A New Model Uses AI to Discover New Materials
A New Model Uses AI to Discover New Materials
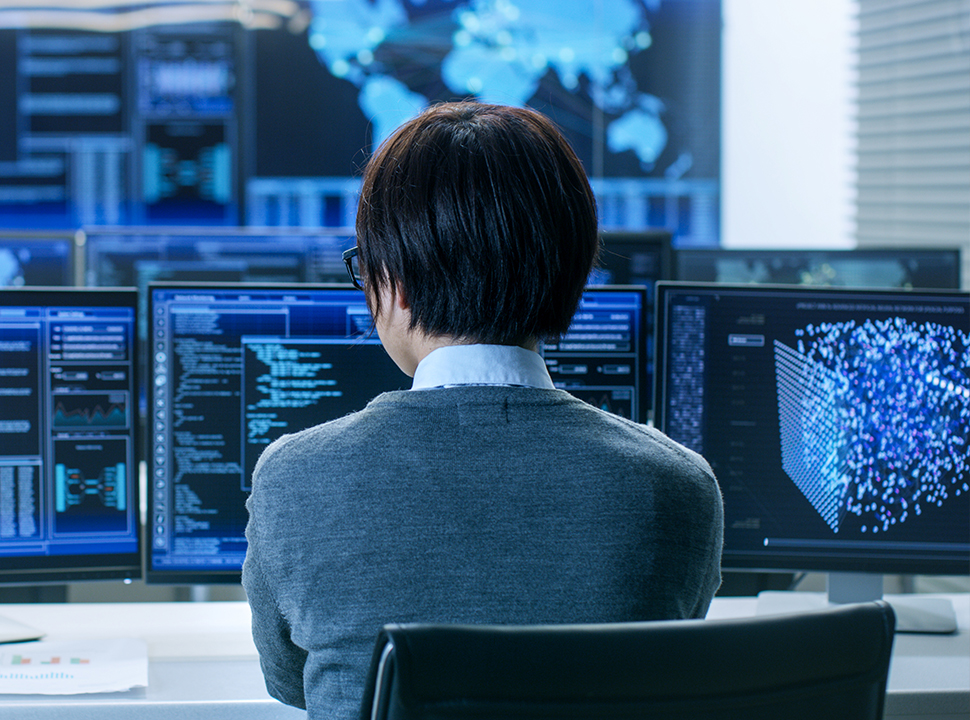
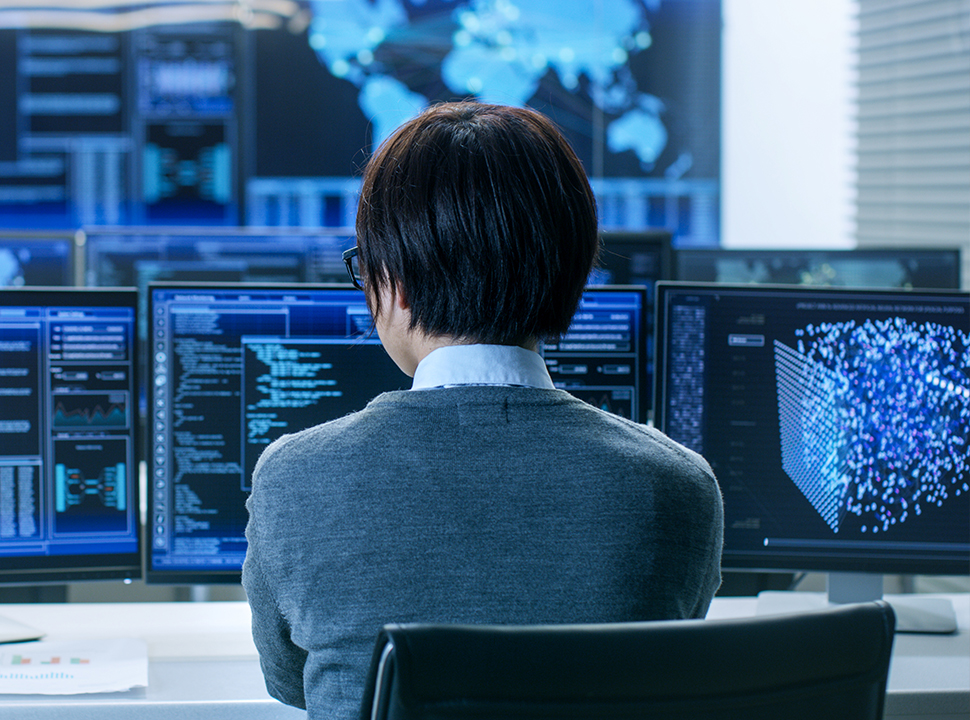
The quest for new, faster and stronger materials of the future are being tested by researchers at University of Illinois, Chicago, whose new model can greatly speed the discovery process.
All-new materials with unique material properties are needed for the ever-faster computers, electric-vehicle batteries, and lighter vehicles—to name just a few of the new inventions and technologies—in our future.
A new computer model from the University of Illinois, Chicago, can greatly speed the discovery process.
New materials might need to be created to specifically withstand high temperatures inside a nuclear reactor or to deflect a significant force in a traffic accident, for example. Electric vehicle batteries need materials durable enough to last 10 years and to be charged thousands of times, according to the National Renewable Energy Laboratory.
But discovering new materials is a time-consuming process that is too often done through trial and error, said Ali Davariashtiyani, a doctoral student at the University of Illinois, Chicago.
More Like This: Implanted Medical Devices Depend on Bioresorbable Materials
During the discovery process, scientists combine atoms in new ways, creating the crystals that will form the basis of the material. Digital models can determine when a new crystal has the right properties and whether it’s stable enough to exist.
But the models couldn’t say whether materials made with the crystals could actually be created in the real world.
Finding out is a slow task, hindered by setbacks. Today, scientists take theoretical shortcuts to predict how changing a given atom in a crystal would change the crystal’s properties and make it stable enough to actually form a material. But the practice limits them to making small changes in already known materials, Davariashtiyani said.
Adding to that, the synthesizability of a crystalline material is governed by many parameters, such as thermodynamic stability, the kinetics of the synthesis process, and synthesis routes (synthesis history), making a one-on-one map of synthesizability to simple physical parameters infeasible, Davariashtiyani said.
His team has created an AI model that helps identify materials that can be naturally formed. It has the potential to slash development time, getting us to resilient electric vehicle batteries and stronger airplanes and automobiles that much faster.
Continue Your Read: Manufacturing Blog: Ceramic Materials Advance Climate Change Efforts
The researchers first used software to encode a crystal’s chemical structure, properties, and ability to be synthesized, and used that information to create a kind of abstract, three-dimensional image. While crystals are represented with 3D images, voxels within the images are color-coded by the chemical attributes of the elements that make them up, Davariashtiyan said.
“Each crystalline material is digitized into a uniquely distinguishable image,” he said.
A person couldn’t create or understand such an image directly; a computer needs to translate it, Davariashtiyan added.
The system learns the features of hidden structural and chemical arrangements of crystalline materials, and the AI feature can quickly tell if they can be synthesized—created in the real world—or not, he explained.
To train the system, Davariashtiyani started with a group of crystals he knew could be synthesized, but that couldn’t necessarily be built in the real world. With the help of a vision recognition system, the model was slowly able to recognize which were and weren’t buildable. Then he tested the AI on another known group of crystals without labeling their properties, to see how accurately it predicted synthesizability.
Related Content: 9 Material Discoveries that Could Transform Manufacturing
Without human intervention, the AI was able to predict whether those crystals could be synthesized in the lab, he said.
The researchers have shown the feasibility of their existing model by accurately predicting the synthesizability of hypothetical crystals for battery electrode and thermoelectric applications, Davariashtiyani said.
Now they’re working to hone their AI model to find buildable crystals that have particular properties and that can be built in particular environments. For instance, he hopes to develop an AI model that can make predictions of how pressure can influence the synthesizability of crystals and a model that can discover crystalline materials with extreme hardness, he added.
Future inventions that are faster, stronger, and more adept than those we have today await.
Jean Thilmany is a science and technology writer in Saint Paul, Minn.
A new computer model from the University of Illinois, Chicago, can greatly speed the discovery process.
New materials might need to be created to specifically withstand high temperatures inside a nuclear reactor or to deflect a significant force in a traffic accident, for example. Electric vehicle batteries need materials durable enough to last 10 years and to be charged thousands of times, according to the National Renewable Energy Laboratory.
But discovering new materials is a time-consuming process that is too often done through trial and error, said Ali Davariashtiyani, a doctoral student at the University of Illinois, Chicago.
More Like This: Implanted Medical Devices Depend on Bioresorbable Materials
During the discovery process, scientists combine atoms in new ways, creating the crystals that will form the basis of the material. Digital models can determine when a new crystal has the right properties and whether it’s stable enough to exist.
But the models couldn’t say whether materials made with the crystals could actually be created in the real world.
Finding out is a slow task, hindered by setbacks. Today, scientists take theoretical shortcuts to predict how changing a given atom in a crystal would change the crystal’s properties and make it stable enough to actually form a material. But the practice limits them to making small changes in already known materials, Davariashtiyani said.
Adding to that, the synthesizability of a crystalline material is governed by many parameters, such as thermodynamic stability, the kinetics of the synthesis process, and synthesis routes (synthesis history), making a one-on-one map of synthesizability to simple physical parameters infeasible, Davariashtiyani said.
His team has created an AI model that helps identify materials that can be naturally formed. It has the potential to slash development time, getting us to resilient electric vehicle batteries and stronger airplanes and automobiles that much faster.
Continue Your Read: Manufacturing Blog: Ceramic Materials Advance Climate Change Efforts
The researchers first used software to encode a crystal’s chemical structure, properties, and ability to be synthesized, and used that information to create a kind of abstract, three-dimensional image. While crystals are represented with 3D images, voxels within the images are color-coded by the chemical attributes of the elements that make them up, Davariashtiyan said.
“Each crystalline material is digitized into a uniquely distinguishable image,” he said.
A person couldn’t create or understand such an image directly; a computer needs to translate it, Davariashtiyan added.
The system learns the features of hidden structural and chemical arrangements of crystalline materials, and the AI feature can quickly tell if they can be synthesized—created in the real world—or not, he explained.
To train the system, Davariashtiyani started with a group of crystals he knew could be synthesized, but that couldn’t necessarily be built in the real world. With the help of a vision recognition system, the model was slowly able to recognize which were and weren’t buildable. Then he tested the AI on another known group of crystals without labeling their properties, to see how accurately it predicted synthesizability.
Related Content: 9 Material Discoveries that Could Transform Manufacturing
Without human intervention, the AI was able to predict whether those crystals could be synthesized in the lab, he said.
The researchers have shown the feasibility of their existing model by accurately predicting the synthesizability of hypothetical crystals for battery electrode and thermoelectric applications, Davariashtiyani said.
Now they’re working to hone their AI model to find buildable crystals that have particular properties and that can be built in particular environments. For instance, he hopes to develop an AI model that can make predictions of how pressure can influence the synthesizability of crystals and a model that can discover crystalline materials with extreme hardness, he added.
Future inventions that are faster, stronger, and more adept than those we have today await.
Jean Thilmany is a science and technology writer in Saint Paul, Minn.
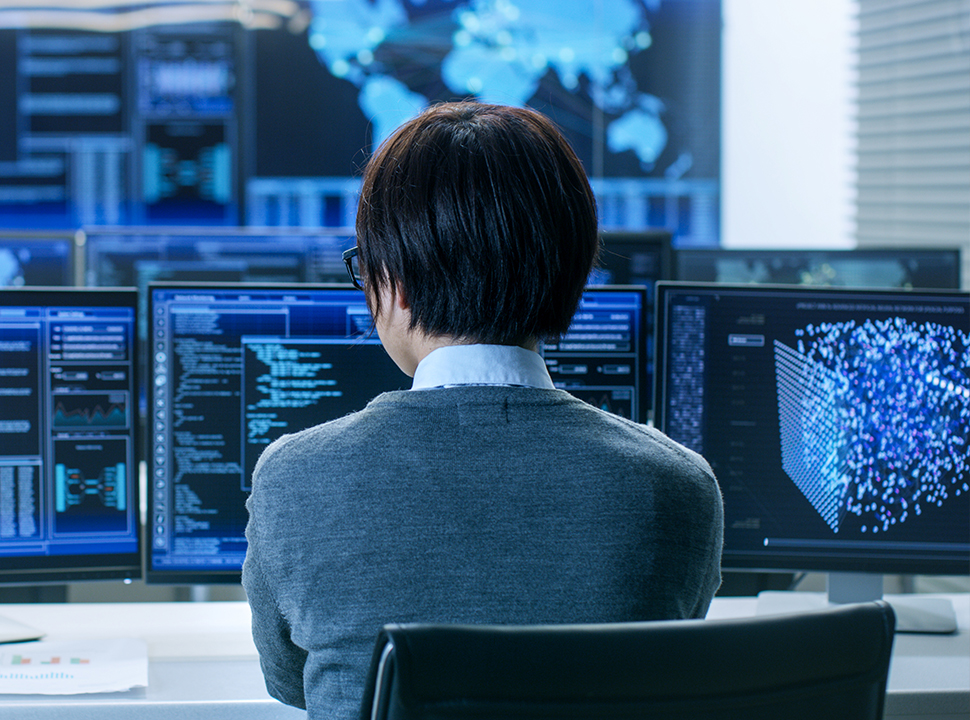
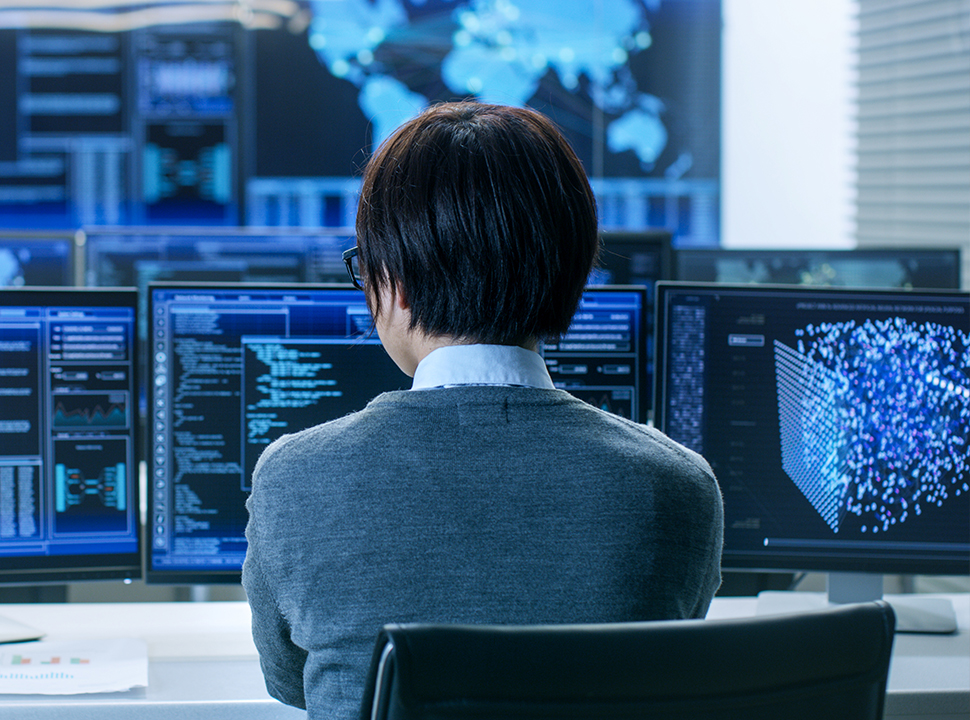