Engineers Learn to Work with AI
Engineers Learn to Work with AI
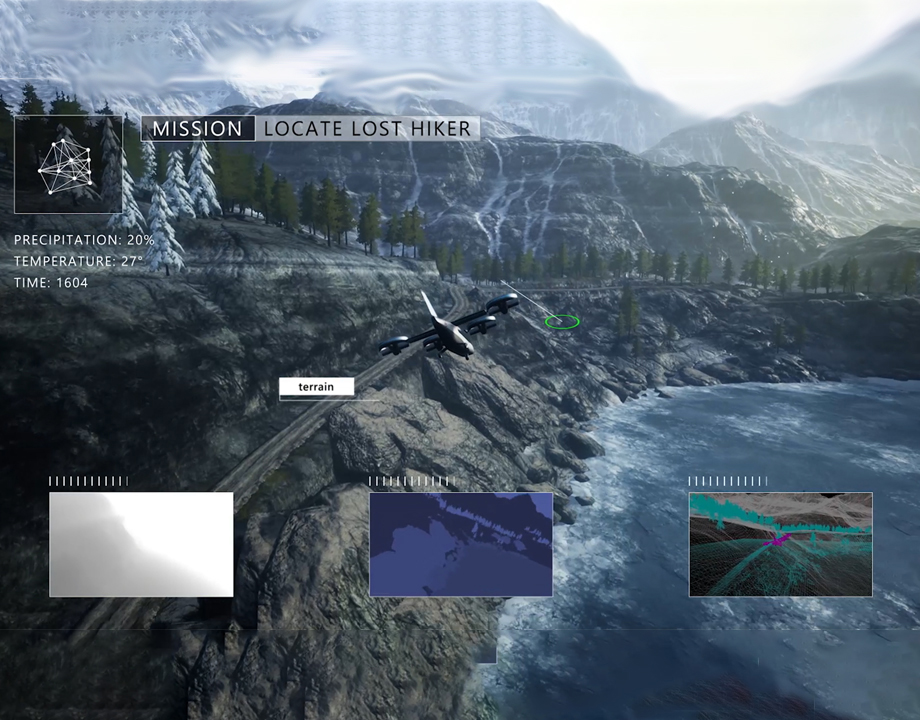
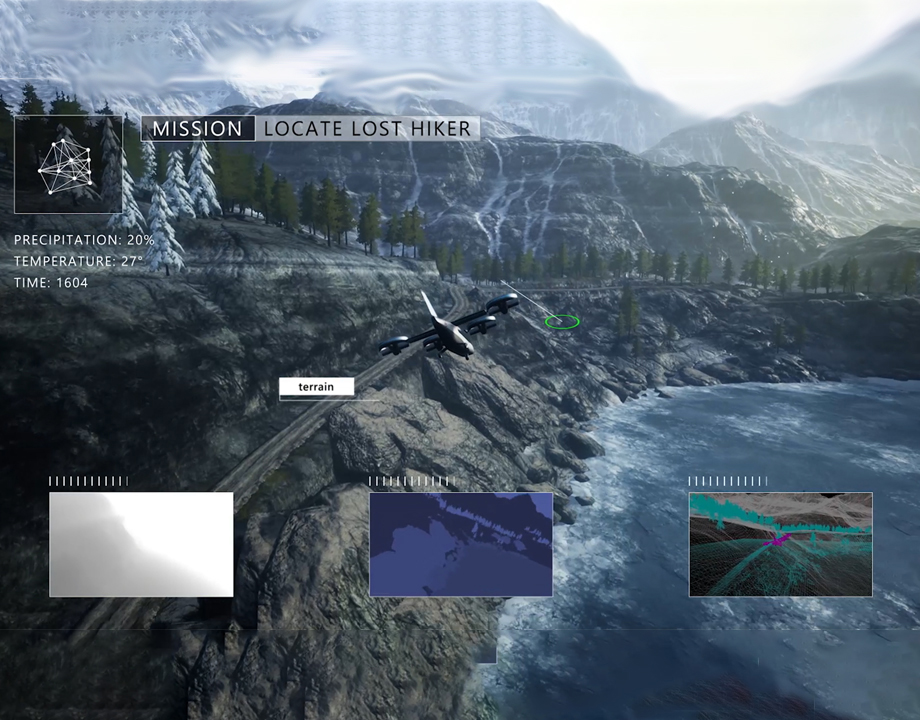
Artificial intelligence is reshaping many popular design and process optimization tools. How can mechanical engineers harness these new algorithms to enhance their skills?
In the not too distant future, a junior engineer will open a computer-aided design program on her laptop and begin to sketch out a simple 2D profile for a new grip mechanism on a manufacturing robot arm. Looking back over her notes from an earlier design meeting, she will start to move the cursor to create the outline of the component. As she does so, the software will make subdued suggestions in gray, based both on the way she has historically worked in the application as well as uploads of previous design profiles for this particular part.
Instead of having to painstakingly enter the geometric constraints and other key design requirements, the application will generate its own idea of where the design is going, even highlighting what it sees as a potential error, based on a large set of simulation data for the full robotic arm this part may one day be placed in. Once the engineer notices that alert, she will quickly find, analyze, and fix the problem, finishing this iteration of the grip design in record time and sending it on to a colleague for review.
That scenario may sound like science fiction, but some of those capabilities may well be in the next version of your favorite CAD program—or even the one you are using right now. Popular CAD platforms, including PTC’s Creo and Siemens NX, have now implemented artificial intelligence (AI) and machine learning (ML) functionality—better known as generative design and topology optimization tools—in their latest releases. These tools offer engineers the ability to more quickly innovate, collaborate, analyze, and optimize their designs.
“There is tremendous opportunity to use AI to unlock the creativity of engineers and supercharge their ability to innovate,” said Gurdeep Pall, corporate vice president of business AI at Microsoft. “Modern systems are inherently getting more complex and AI is perfectly suited to tackle complexity.”
Learn More About Digital Engineering: ASME Digital Twin Summit
But the addition of AI/ML is not limited solely to the design space. Many of the large software giants, including Microsoft and Google, are betting that such algorithms can also drive unparalleled improvements in various manufacturing processes, too. And that raises a vital question: As AI/ML solutions become more pervasive in different areas of the mechanical engineering arena, how can mechanical engineers learn how to best utilize them for success?
Enhancing Design
AI, or computing systems designed to accomplish tasks that usually require human intelligence, like reasoning, decision-making, problem-solving, and learning, are now being used across a wide swath of industries. You have likely seen just what ML, or a type of AI algorithm that uses large data sets to fuel learning, can do the last time you logged into Amazon or Spotify and they made a specific recommendation based on your use history. But AI/ML’s advantages aren’t limited to the consumer applications—they also hold great promise in engineering and design.
CAD platforms have long been an invaluable tool in the mechanical engineer’s arsenal, allowing engineers the ability to design and review potential design solutions, making those vital trade-offs between cost and performance, before committing to a build. Yet, as functionality has increased, so has the ability to efficiently use such tools to their full potential.
“CAD tools are now quite mature and have all kinds of different commands and operations that you can perform in them, which is of benefit to engineers and their designs,” said Chad Jackson, chief analyst & CEO of Lifecycle Insights. “But we’re now at the point where there are 500 different commands you could execute as you design a circuit board or mechanical piece of hardware. It’s almost become too much.”
This is exactly where AI can help, said Paul Bevan, a senior technical product manager at Siemens, and design engineer by training. The right ML algorithm could learn the standard workflows that certain individuals or organizations typically use, transforming the user interface at different steps to provide a toolbar with a focused set of commands. That’s why Siemens has implemented such an algorithm in its widely-used NX CAD system.
“The algorithm is able to see that, most of the time, once you complete this step, you usually go on to this particular command, so it gives you the most commonly used commands based on typical workflows,” Bevan said. “It can quickly adapt based on a user’s behavior and it can predict, with about 95 percent accuracy, what commands people want to use at that point to continue on with that design.”
Creo, PTC’s CAD offering, has added generative design capabilities, as well as simulation-driven design, to help engineers more quickly produce optimized designs for engineered systems. Jesse Coors-Blankenship, senior vice president of technology at PTC, said AI-related improvements can help younger engineers be more productive and create better designs faster.
“Some engineers may not have the real-world experience yet to know what designs are best to solve a particular problem,” he said. “This approach allows engineers to get designs automatically generated by the requirements they specify. It is also architected in a way so it can effectively handle any change requirements during the entire design process. So, instead of weeding through and testing hundreds of designs, it can help you get to a handful of good designs in a relatively short period of time, allow you to easily iterate those designs, and speed up the whole development process.”
Editor's Choice: Podcast: The Expanding Role of Digital Engineering in Manufacturing
Designs developed with the help of AI/ML may not end up looking the way you thought they might. Todd Grimm, president of T. A. Grimm & Associates and an expert in additive manufacturing, said that Siemens, in partnership with HP 3D Printing, just used the NX system to optimize the additive manufacturing design of a printer cooling duct. Using NX, with input from computational fluid dynamic simulations, the engineering team came up with design that looks a bit like an old-school Dustbuster vacuum with a tongue flopping out of its mouth. Yet, this bizarre looking result offered a 22 percent performance improvement over the old part.
“These platforms are meant to be innovative idea generators and can allow engineers to think beyond what they can conceive of with just a blank sheet of paper,” Grimm said. “When you look at the end design of this part, it seems counterintuitive to the design that most engineers would think would work best. These new tools have the potential to really open up the design space to places we might not have gotten to on our own.”
Beyond Design
Coors-Blankenship said that AI can optimize other engineering processes as well. He and his team are currently working on AI upgrades that will help to support concurrent engineering and collaborative work. Such product data management programs can not only control who has access to design files—and, even, perhaps, specific parts of design files—but also keeps track of who changed what when.
“One engineer may have really good knowledge regarding the physical requirements of product performance, another may have more experience in manufacturing-related processes,” he said. “This kind of solution can support a team coming together into a single, aggregate data source to create a generative model that can evolve and adapt based on all the different information it receives. This kind of approach helps to save not only time, but the other costs surrounding procurement, materials, and manufacturing needs.”
Those benefits could help with manufacturing optimization, as well. Microsoft’s Pall is currently heading up Project Bonsai, a new program that uses AI to help engineers create and optimize autonomous control systems in manufacturing. In the past, creating the right approach could take quite a bit of time, Pall said, and even more trial and error.
“Today, simulations are primarily used for validation and testing,” he said. “But simulators can also provide the synthetic data needed for an AI to learn. As such, developing a faithful representation of the physics, mechanics, chemistry, and other factors needed to model the system behavior can help provide information to accelerate innovation, increase the speed of production, and reduce waste. The AI model can run a particular simulation 100,000 times in a day so, instead of it taking several months to come up with the optimization, it can be done in a week.”
There are dozens of other AI/ML use cases for manufacturing optimization and factory automation. Some systems may suggest parts that a company already makes or buys to help reduce warehouse or manufacturing costs. Others can give production estimates based on in-house machinery. Still others can provide assistance with quality assurance management. For example, Siemens is currently working on AI/ML solutions that can provide predictive maintenance information for the factory floor.
Read More: Digital Engineering During COVID-19
“The machines we use in manufacturing are quite expensive – and if they fail, you usually have to halt production,” said Colm Gavin, a digitization specialist at Siemens. “There could be a lot of wear and tear on the bearings, or perhaps someone accidentally leaves a screwdriver near the mold that could cause a problem. But if an AI solution could watch and listen to what’s happening to identify potential problems, you can predict an issue before the machine fails and just take care of it, saving a lot of time and money in the process.”
Put it all together and it soon becomes clear that AI/ML engineering solutions are growing—and growing quickly across the entirety of the field. Allen Robinson, a mechanical engineering professor at Carnegie Mellon University, said it’s high time that engineers stop thinking of AI/ML solutions as something that will occur in the future.
“This isn’t something that is coming,” he said. “This is something that is already here. Many people don’t realize that AI/ML, as well as more data-driven design and manufacturing approaches, are already having a big impact on the way engineering is done and how engineers do their work. It’s not going away.”
Working with AI/ML
As data-driven models become more ubiquitous across all industries, it’s important that engineers are prepared to work with AI/ML technologies in a way that augments their existing knowledge and abilities. Given that developers like Coors-Blankenship and Bevan are working hard to ensure that any AI/ML enhancements seamlessly work in the background of their design tools, one might think engineers can simply just keep doing their work the way they always have—the algorithm will just fill in the blanks for you. But that’s not necessarily the case.
Any ML algorithm is only as good as the data one puts into it—so Robinson said it is vital that engineers are prepared to learn how to become sophisticated users of such models. Carnegie Mellon and other highly rated technical universities offer AI/ML courses for mechanical engineers at both the undergraduate and graduate levels.
“We are trying to teach students how AI/ML can complement the more traditional physics-based design principles that every engineer should know,” he said. “We are trying to show them how they can apply these tools to get to the best design—but getting to the best design means they need to understand the essence of the problem. If your AI/ML algorithm has been trained on data that doesn’t overlap your problem space, you aren’t going to get a solution that works.”
A generative design tool, for example, might lead to a faulty design if the constraints aren’t properly inputted. Robinson said that mechanical engineers should understand the problem space well enough to identify when the model has gone astray.
Gavin, for his part, said he doesn’t expect mechanical engineers to become data scientists so they can more effectively use AI/ML solutions. Rather, they can and should be the subject matter experts that provide the appropriate data to create fruitful AI models.
“There may be a few mechanical engineers who end up writing AI algorithms, if that is where their interest lies,” he said. “But I think it is more important that they really understand the principles underlying the problem the AI is trying to solve so they can provide that knowledge to the data scientist or programmer who will build the model. If a mechanical engineer designed a particular machine, they know at what points it may be most likely fail, what kinds of noises or vibrations are the most problematic. They can give that information to the programmer to make sure the programmer can create an algorithm that will actually work.”
The data scientists who are building the AI/ML tools of the future believe that engineers can best work with such models by simply being the best engineers they can be—fully understanding the mechanical constraints of any problem inside-out. But Bevan added that one of the best things that engineers can do right now to prepare for a more AI/ML-filled future is simply being willing to keep an open mind about what they might offer and accept the changes as they come.
Take the Quiz: How Much of a Digital Engineer Are You?
“I first started in the industry designing machinery and the tools have changed quite a bit since then,” he said. “These algorithms can add a lot to design. There’s no reason to just stick to the status quo just because that’s the way we’ve always done things. I hope that people are willing to accept some of these changes and see we are just providing a broader set of tools that give engineers a chance to create better solutions.”
And as for the old trope about AI/ML models one day replacing mechanical engineers? Grimm said the idea is utter nonsense. AI/ML algorithms can augment an engineer’s abilities but they don’t have the power to replace them.
“AI/ML is not something that engineers should be afraid of,” Grimm said. “Rather, it should be viewed as an advance that liberates us and takes away some of the drudgery and grunt work. It’s not something will take away your creativity as a designer. It should actually enhance it, especially if the AI/ML suggests something that then takes you down a path you might not have otherwise considered. But, as with anything else in engineering, you have to apply your knowledge, intelligence, and experience to make that new thing possible. It’s only going to go where you lead it.”
Kayt Sukel is a technology journalist based in Houston.
Instead of having to painstakingly enter the geometric constraints and other key design requirements, the application will generate its own idea of where the design is going, even highlighting what it sees as a potential error, based on a large set of simulation data for the full robotic arm this part may one day be placed in. Once the engineer notices that alert, she will quickly find, analyze, and fix the problem, finishing this iteration of the grip design in record time and sending it on to a colleague for review.
That scenario may sound like science fiction, but some of those capabilities may well be in the next version of your favorite CAD program—or even the one you are using right now. Popular CAD platforms, including PTC’s Creo and Siemens NX, have now implemented artificial intelligence (AI) and machine learning (ML) functionality—better known as generative design and topology optimization tools—in their latest releases. These tools offer engineers the ability to more quickly innovate, collaborate, analyze, and optimize their designs.
“There is tremendous opportunity to use AI to unlock the creativity of engineers and supercharge their ability to innovate,” said Gurdeep Pall, corporate vice president of business AI at Microsoft. “Modern systems are inherently getting more complex and AI is perfectly suited to tackle complexity.”
Learn More About Digital Engineering: ASME Digital Twin Summit
But the addition of AI/ML is not limited solely to the design space. Many of the large software giants, including Microsoft and Google, are betting that such algorithms can also drive unparalleled improvements in various manufacturing processes, too. And that raises a vital question: As AI/ML solutions become more pervasive in different areas of the mechanical engineering arena, how can mechanical engineers learn how to best utilize them for success?
Enhancing Design
AI, or computing systems designed to accomplish tasks that usually require human intelligence, like reasoning, decision-making, problem-solving, and learning, are now being used across a wide swath of industries. You have likely seen just what ML, or a type of AI algorithm that uses large data sets to fuel learning, can do the last time you logged into Amazon or Spotify and they made a specific recommendation based on your use history. But AI/ML’s advantages aren’t limited to the consumer applications—they also hold great promise in engineering and design.
CAD platforms have long been an invaluable tool in the mechanical engineer’s arsenal, allowing engineers the ability to design and review potential design solutions, making those vital trade-offs between cost and performance, before committing to a build. Yet, as functionality has increased, so has the ability to efficiently use such tools to their full potential.
“CAD tools are now quite mature and have all kinds of different commands and operations that you can perform in them, which is of benefit to engineers and their designs,” said Chad Jackson, chief analyst & CEO of Lifecycle Insights. “But we’re now at the point where there are 500 different commands you could execute as you design a circuit board or mechanical piece of hardware. It’s almost become too much.”
This is exactly where AI can help, said Paul Bevan, a senior technical product manager at Siemens, and design engineer by training. The right ML algorithm could learn the standard workflows that certain individuals or organizations typically use, transforming the user interface at different steps to provide a toolbar with a focused set of commands. That’s why Siemens has implemented such an algorithm in its widely-used NX CAD system.
“The algorithm is able to see that, most of the time, once you complete this step, you usually go on to this particular command, so it gives you the most commonly used commands based on typical workflows,” Bevan said. “It can quickly adapt based on a user’s behavior and it can predict, with about 95 percent accuracy, what commands people want to use at that point to continue on with that design.”
Creo, PTC’s CAD offering, has added generative design capabilities, as well as simulation-driven design, to help engineers more quickly produce optimized designs for engineered systems. Jesse Coors-Blankenship, senior vice president of technology at PTC, said AI-related improvements can help younger engineers be more productive and create better designs faster.
“Some engineers may not have the real-world experience yet to know what designs are best to solve a particular problem,” he said. “This approach allows engineers to get designs automatically generated by the requirements they specify. It is also architected in a way so it can effectively handle any change requirements during the entire design process. So, instead of weeding through and testing hundreds of designs, it can help you get to a handful of good designs in a relatively short period of time, allow you to easily iterate those designs, and speed up the whole development process.”
Editor's Choice: Podcast: The Expanding Role of Digital Engineering in Manufacturing
Designs developed with the help of AI/ML may not end up looking the way you thought they might. Todd Grimm, president of T. A. Grimm & Associates and an expert in additive manufacturing, said that Siemens, in partnership with HP 3D Printing, just used the NX system to optimize the additive manufacturing design of a printer cooling duct. Using NX, with input from computational fluid dynamic simulations, the engineering team came up with design that looks a bit like an old-school Dustbuster vacuum with a tongue flopping out of its mouth. Yet, this bizarre looking result offered a 22 percent performance improvement over the old part.
“These platforms are meant to be innovative idea generators and can allow engineers to think beyond what they can conceive of with just a blank sheet of paper,” Grimm said. “When you look at the end design of this part, it seems counterintuitive to the design that most engineers would think would work best. These new tools have the potential to really open up the design space to places we might not have gotten to on our own.”
Beyond Design
Coors-Blankenship said that AI can optimize other engineering processes as well. He and his team are currently working on AI upgrades that will help to support concurrent engineering and collaborative work. Such product data management programs can not only control who has access to design files—and, even, perhaps, specific parts of design files—but also keeps track of who changed what when.
“One engineer may have really good knowledge regarding the physical requirements of product performance, another may have more experience in manufacturing-related processes,” he said. “This kind of solution can support a team coming together into a single, aggregate data source to create a generative model that can evolve and adapt based on all the different information it receives. This kind of approach helps to save not only time, but the other costs surrounding procurement, materials, and manufacturing needs.”
Those benefits could help with manufacturing optimization, as well. Microsoft’s Pall is currently heading up Project Bonsai, a new program that uses AI to help engineers create and optimize autonomous control systems in manufacturing. In the past, creating the right approach could take quite a bit of time, Pall said, and even more trial and error.
“Today, simulations are primarily used for validation and testing,” he said. “But simulators can also provide the synthetic data needed for an AI to learn. As such, developing a faithful representation of the physics, mechanics, chemistry, and other factors needed to model the system behavior can help provide information to accelerate innovation, increase the speed of production, and reduce waste. The AI model can run a particular simulation 100,000 times in a day so, instead of it taking several months to come up with the optimization, it can be done in a week.”
There are dozens of other AI/ML use cases for manufacturing optimization and factory automation. Some systems may suggest parts that a company already makes or buys to help reduce warehouse or manufacturing costs. Others can give production estimates based on in-house machinery. Still others can provide assistance with quality assurance management. For example, Siemens is currently working on AI/ML solutions that can provide predictive maintenance information for the factory floor.
Read More: Digital Engineering During COVID-19
“The machines we use in manufacturing are quite expensive – and if they fail, you usually have to halt production,” said Colm Gavin, a digitization specialist at Siemens. “There could be a lot of wear and tear on the bearings, or perhaps someone accidentally leaves a screwdriver near the mold that could cause a problem. But if an AI solution could watch and listen to what’s happening to identify potential problems, you can predict an issue before the machine fails and just take care of it, saving a lot of time and money in the process.”
Put it all together and it soon becomes clear that AI/ML engineering solutions are growing—and growing quickly across the entirety of the field. Allen Robinson, a mechanical engineering professor at Carnegie Mellon University, said it’s high time that engineers stop thinking of AI/ML solutions as something that will occur in the future.
“This isn’t something that is coming,” he said. “This is something that is already here. Many people don’t realize that AI/ML, as well as more data-driven design and manufacturing approaches, are already having a big impact on the way engineering is done and how engineers do their work. It’s not going away.”
Working with AI/ML
As data-driven models become more ubiquitous across all industries, it’s important that engineers are prepared to work with AI/ML technologies in a way that augments their existing knowledge and abilities. Given that developers like Coors-Blankenship and Bevan are working hard to ensure that any AI/ML enhancements seamlessly work in the background of their design tools, one might think engineers can simply just keep doing their work the way they always have—the algorithm will just fill in the blanks for you. But that’s not necessarily the case.
Any ML algorithm is only as good as the data one puts into it—so Robinson said it is vital that engineers are prepared to learn how to become sophisticated users of such models. Carnegie Mellon and other highly rated technical universities offer AI/ML courses for mechanical engineers at both the undergraduate and graduate levels.
“We are trying to teach students how AI/ML can complement the more traditional physics-based design principles that every engineer should know,” he said. “We are trying to show them how they can apply these tools to get to the best design—but getting to the best design means they need to understand the essence of the problem. If your AI/ML algorithm has been trained on data that doesn’t overlap your problem space, you aren’t going to get a solution that works.”
A generative design tool, for example, might lead to a faulty design if the constraints aren’t properly inputted. Robinson said that mechanical engineers should understand the problem space well enough to identify when the model has gone astray.
Gavin, for his part, said he doesn’t expect mechanical engineers to become data scientists so they can more effectively use AI/ML solutions. Rather, they can and should be the subject matter experts that provide the appropriate data to create fruitful AI models.
“There may be a few mechanical engineers who end up writing AI algorithms, if that is where their interest lies,” he said. “But I think it is more important that they really understand the principles underlying the problem the AI is trying to solve so they can provide that knowledge to the data scientist or programmer who will build the model. If a mechanical engineer designed a particular machine, they know at what points it may be most likely fail, what kinds of noises or vibrations are the most problematic. They can give that information to the programmer to make sure the programmer can create an algorithm that will actually work.”
The data scientists who are building the AI/ML tools of the future believe that engineers can best work with such models by simply being the best engineers they can be—fully understanding the mechanical constraints of any problem inside-out. But Bevan added that one of the best things that engineers can do right now to prepare for a more AI/ML-filled future is simply being willing to keep an open mind about what they might offer and accept the changes as they come.
Take the Quiz: How Much of a Digital Engineer Are You?
“I first started in the industry designing machinery and the tools have changed quite a bit since then,” he said. “These algorithms can add a lot to design. There’s no reason to just stick to the status quo just because that’s the way we’ve always done things. I hope that people are willing to accept some of these changes and see we are just providing a broader set of tools that give engineers a chance to create better solutions.”
And as for the old trope about AI/ML models one day replacing mechanical engineers? Grimm said the idea is utter nonsense. AI/ML algorithms can augment an engineer’s abilities but they don’t have the power to replace them.
“AI/ML is not something that engineers should be afraid of,” Grimm said. “Rather, it should be viewed as an advance that liberates us and takes away some of the drudgery and grunt work. It’s not something will take away your creativity as a designer. It should actually enhance it, especially if the AI/ML suggests something that then takes you down a path you might not have otherwise considered. But, as with anything else in engineering, you have to apply your knowledge, intelligence, and experience to make that new thing possible. It’s only going to go where you lead it.”
Kayt Sukel is a technology journalist based in Houston.