Manufacturing Blog: Integrating AI into Production Processes
Manufacturing Blog: Integrating AI into Production Processes
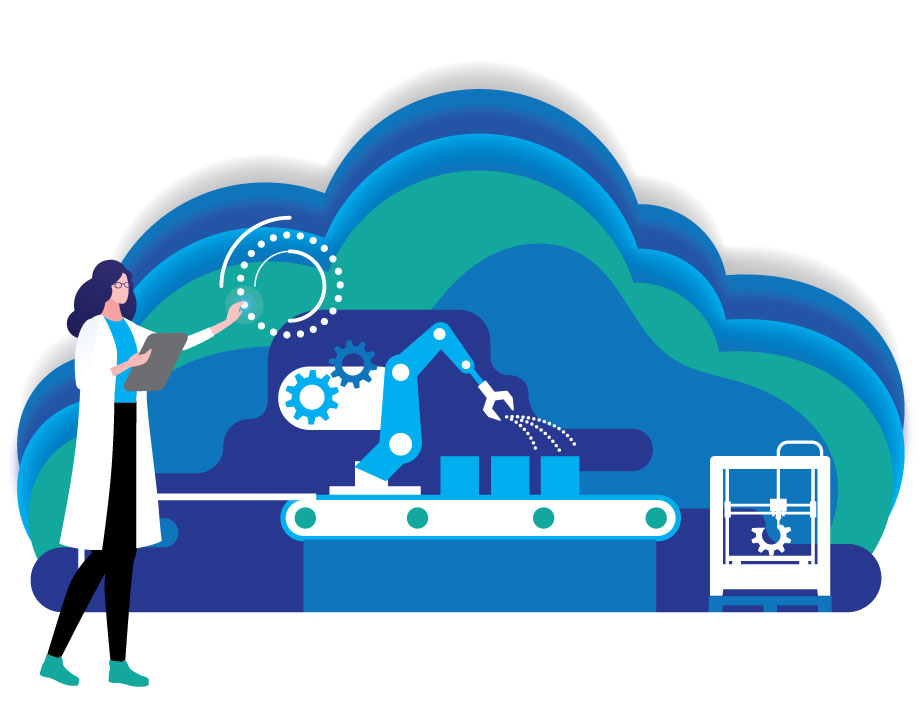
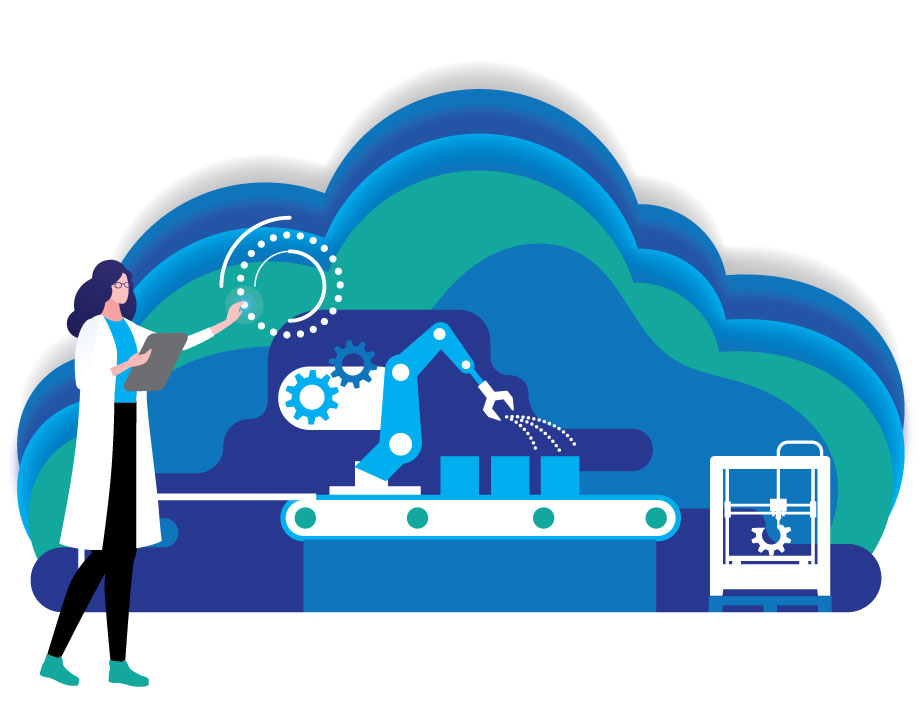
AI is becoming an important tool, but initial costs and lack of universal data prevent smaller firms from deploying it, at least for now.
U.S. manufacturing is awash in new money, as the legislative package passed in 2021 and 2022 targeting infrastructure, clean energy, and the semiconductor industry begins to distribute investment money and tax incentives. According to a 2024 outlook of the manufacturing sector recently released by Deloitte, the legislation has already leveraged record private sector investment in manufacturing.
For instance, some 200 new clean technology manufacturing plants have been announced since the passage of the Inflation Reduction Act, representing $88 billion in investment. Semiconductor and clean technology manufacturing have seen investment jump nearly 20 times from 2019 levels.
But Deloitte sees problems looming in the coming year. The continuing shortage of skilled labor, economic uncertainty, and challenges drive by the need for product innovation to meet net-zero emissions goals could hamper the industry. Technology is expected to be a major tool in combatting those problems. Deloitte points to surveys showing 86 percent of manufacturing executives think smart factories will drive competitiveness, and the industrial metaverse could point the way to a 12 percent gain in labor productivity.
Become a Member: How to Join ASME
Generative AI will also play important roles in product design and supply chain management and could help reduce labor costs. “Now that many manufacturers have gone through significant transformations to keep assets running efficiently and minimize reductions in output, they are shifting focus to meet consumer demand for customized goods,” according to the report.
That requires a nimbler operation. Amazon, for instance, is investing heavily in robots enhanced with AI to improve warehouse organization and product distribution.
The company’s Sequoia robotic system identifies and stores inventory at fulfillment centers up to 75 percent faster than previously, claims the firm. After an order is placed, it also reduces the processing time up to 25 percent, improving shipping predictability.
Sequoia integrates multiple robotic systems, including mobile robots, gantry cranes, robotic arms, and ergonomic workstations. According to the company, mobile robots transport containerized inventory directly to a gantry that can either restock the product, or “tote,” or send to a human employee to pick out the ordered item. New workstations provide a working area between mid-thigh and mid-chest height, a height that should reduce worker injuries from stretching and lifting.
More for You: Automation and Robotics Increase Footprint Across Manufacturing
Amazon works with Agility Robotics to test its bipedal robot Digit that can move, grasp, and handle items in corners and other spaces. It will initially be used for repetitive tasks such as tote recycling, basically picking up and moving empty containers. Computer vision and speech recognition are expected to further meld with robotics to advance these examples.
AI and deep learning models have been slow to come to manufacturing for two reasons, according to the MIT Sloan School of Management. Prohibitive costs have kept it from all but the largest manufacturers, and a lack of universal industrial data slows adoption. Data can be specific to a certain industry or even a specific company, resulting in a dearth of relevant data.
"There’s no such thing for manufacturing operations―there is no universal availability of data from turbines, cars, other signals that we are capturing,” said Kalyan Veeramachaneni, principal research scientist at MIT’s Schwarzman College of Computing at a recent conference.
MIT researchers believe generative AI and data-centric AI make it more accessible. Tools such as ChatGPT provide a more intuitive way to model complex data and images, opening the technology to a broader user base. Data-centric AI shifts the focus away from specialized algorithms to building data sets on which to train an AI system, putting the technology in an understandable form for plant workers or engineers.
The Deloitte survey points out that companies are facing new challenges of working through decarbonization and the integration of AI. Technical readiness, high costs of transitioning production processes, and managing old and new supply chains are a few. The work is just beginning.
John Kosowatz is senior editor.
For instance, some 200 new clean technology manufacturing plants have been announced since the passage of the Inflation Reduction Act, representing $88 billion in investment. Semiconductor and clean technology manufacturing have seen investment jump nearly 20 times from 2019 levels.
But Deloitte sees problems looming in the coming year. The continuing shortage of skilled labor, economic uncertainty, and challenges drive by the need for product innovation to meet net-zero emissions goals could hamper the industry. Technology is expected to be a major tool in combatting those problems. Deloitte points to surveys showing 86 percent of manufacturing executives think smart factories will drive competitiveness, and the industrial metaverse could point the way to a 12 percent gain in labor productivity.
Become a Member: How to Join ASME
Generative AI will also play important roles in product design and supply chain management and could help reduce labor costs. “Now that many manufacturers have gone through significant transformations to keep assets running efficiently and minimize reductions in output, they are shifting focus to meet consumer demand for customized goods,” according to the report.
That requires a nimbler operation. Amazon, for instance, is investing heavily in robots enhanced with AI to improve warehouse organization and product distribution.
The company’s Sequoia robotic system identifies and stores inventory at fulfillment centers up to 75 percent faster than previously, claims the firm. After an order is placed, it also reduces the processing time up to 25 percent, improving shipping predictability.
Sequoia integrates multiple robotic systems, including mobile robots, gantry cranes, robotic arms, and ergonomic workstations. According to the company, mobile robots transport containerized inventory directly to a gantry that can either restock the product, or “tote,” or send to a human employee to pick out the ordered item. New workstations provide a working area between mid-thigh and mid-chest height, a height that should reduce worker injuries from stretching and lifting.
More for You: Automation and Robotics Increase Footprint Across Manufacturing
Amazon works with Agility Robotics to test its bipedal robot Digit that can move, grasp, and handle items in corners and other spaces. It will initially be used for repetitive tasks such as tote recycling, basically picking up and moving empty containers. Computer vision and speech recognition are expected to further meld with robotics to advance these examples.
AI and deep learning models have been slow to come to manufacturing for two reasons, according to the MIT Sloan School of Management. Prohibitive costs have kept it from all but the largest manufacturers, and a lack of universal industrial data slows adoption. Data can be specific to a certain industry or even a specific company, resulting in a dearth of relevant data.
"There’s no such thing for manufacturing operations―there is no universal availability of data from turbines, cars, other signals that we are capturing,” said Kalyan Veeramachaneni, principal research scientist at MIT’s Schwarzman College of Computing at a recent conference.
MIT researchers believe generative AI and data-centric AI make it more accessible. Tools such as ChatGPT provide a more intuitive way to model complex data and images, opening the technology to a broader user base. Data-centric AI shifts the focus away from specialized algorithms to building data sets on which to train an AI system, putting the technology in an understandable form for plant workers or engineers.
The Deloitte survey points out that companies are facing new challenges of working through decarbonization and the integration of AI. Technical readiness, high costs of transitioning production processes, and managing old and new supply chains are a few. The work is just beginning.
John Kosowatz is senior editor.
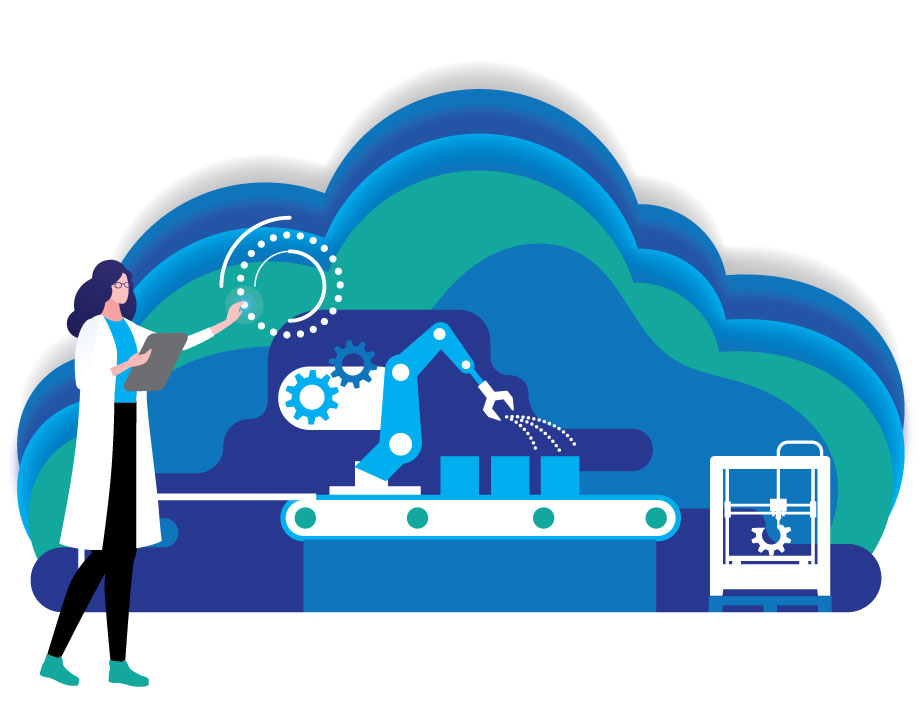
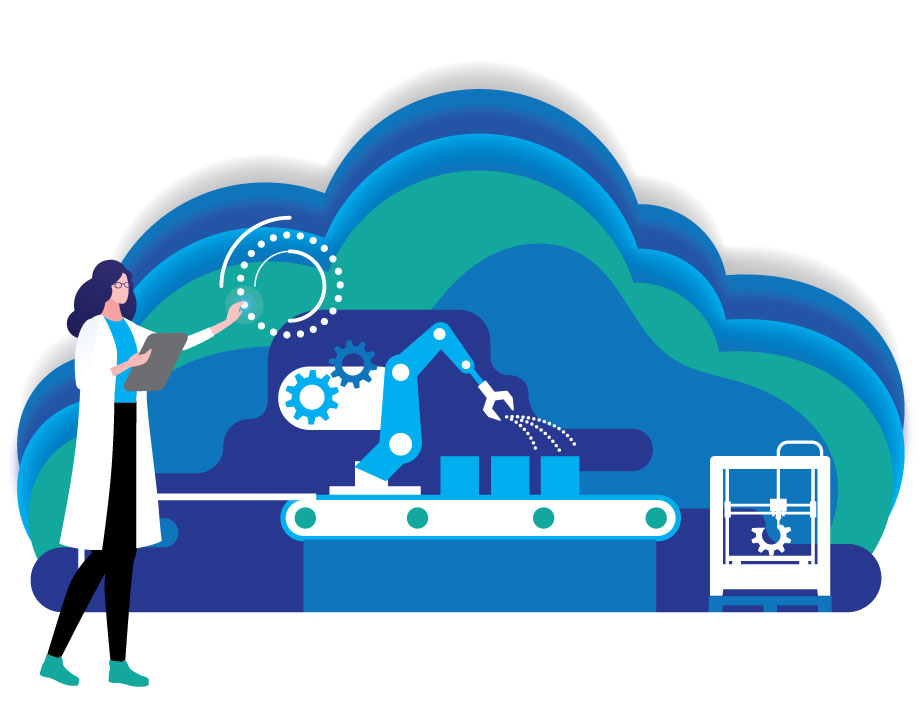