Manufacturing Blog: How to Identify AI Systems and Realistic Implementation
Manufacturing Blog: How to Identify AI Systems and Realistic Implementation
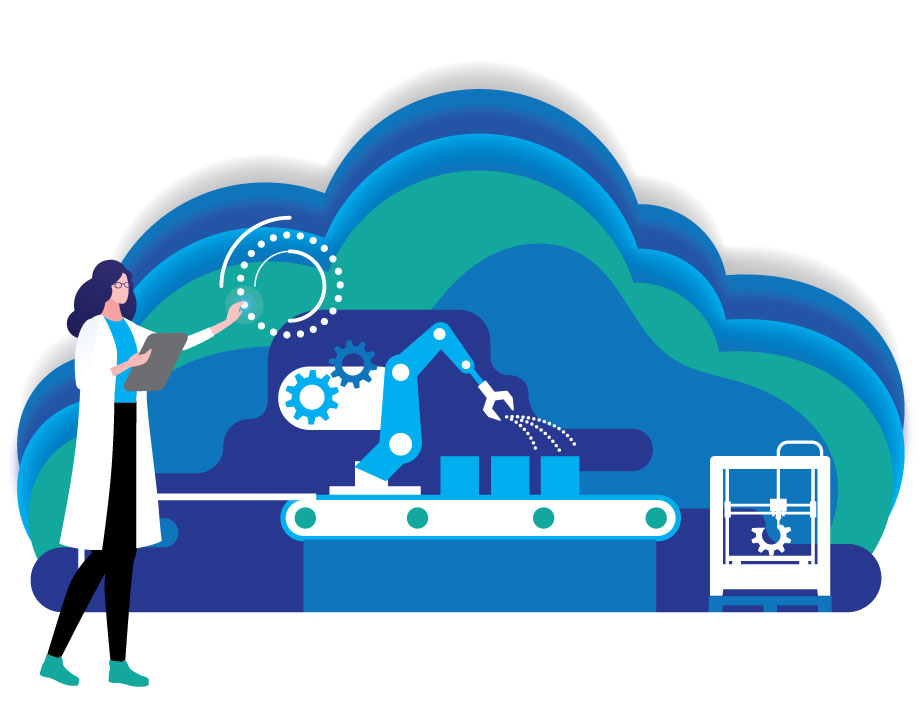
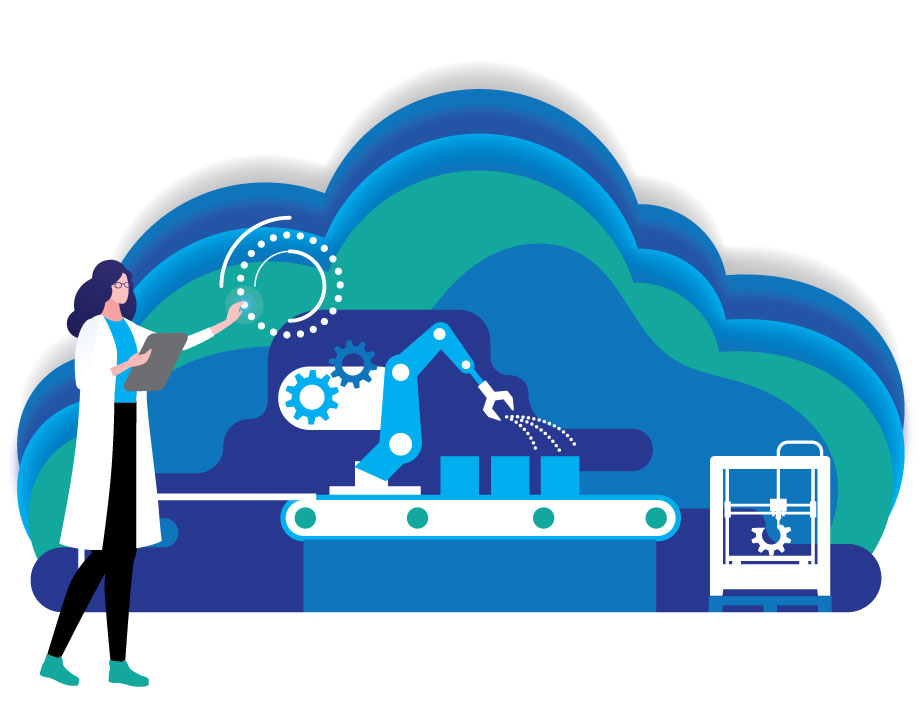
Artificial intelligence systems look to revolutionize manufacturing processes. Setting realistic goals and timelines will aid companies on the road to AI adoption.
Artificial intelligence is becoming an all-encompassing word that is infiltrating every technology under the sun. Companies within the design and manufacturing sector see AI as the next evolving factor, fundamentally changing the manufacturing process.
Adnan Masood, Ph.D. and chief architect of artificial intelligence and machine learning at UST, recently listed all the technologies within manufacturing that look to benefit from AI.
“Beyond hypes and fads, AI works because it amasses significant benefits for the manufacturing sector, such as enabling smart production, developing predictive and preventative maintenance, offering supply chain optimization, improved safety, product development, and optimization, facilitating AR/VR (Augmented and Virtual Reality), cost reduction, quality assurance and enabling green operations (energy management), to name a few,” he wrote for IntelligentCio.com.
With AI being able to impact so many different aspects, it isn’t easy to understand how it will transform manufacturing processes realistically.
Recommended for You: AI and Optimization: A Meeting of the Minds
PwC released “An Introduction to Implementing AI in Manufacturing” in 2020 to help lay out how AI can be deployed across different companies. According to PWC, there are four types of AI:
Implementing AI systems is a fundamental change to an organization’s manufacturing process. It is not as simple as buying a software package and installing it on your computer network. It requires investment and careful planning, from the operational tools to data acquisition and even the types of skilled workers a company hires. According to PWC, there are six building blocks for implementing AI successfully regardless of where you are in the AI process.
The main starting point for an AI strategy is to understand the near-, mid-, and long-term goals. Businesses should group their use cases across all operations into these categories based on function and priority. Identifying low-hanging fruit and obtaining early success will help determine the larger potential of AI and how to plan a road path toward broader implementation.
You May Also Like: 6 AI and Machine Learning Tools for Engineers
The collection of data is key to driving AI systems. However, the key is that data be reliable and accurate, not just a mass collection of information, an aspect of data analysis that plagued early Industry 4.0 systems. The benefit of AI and machine learning is that when data is collected properly, the software can analyze and interpret the data for human workers. Advanced systems can even provide relevant input when making decisions. Large data-management systems require proper cataloging and lineage for tracking purposes and accessibility. Manufacturers need to understand the volume and velocity of data to map their manufacturing processes properly.
PwC points out that the technology for AI systems is rapidly evolving and has yet to reach a mature state. This will cause companies to hesitate from investing in AI systems. However, PwC argues that investing in “functional reference architecture”—the very basics of AI systems—will help companies gain footing and allow them to evolve with AI systems. Some of these essential systems include data management software and cloud-computing services.
When Industry 4.0 processes started to make waves among manufacturing companies, the lack of data scientists to support advanced manufacturing systems impacted its growth. Since then, many organizations have hired data scientists to help make sense of the data collected, but AI systems will require an entirely new team of engineers. According to the PWC, data scientists need to be part of a team that includes data engineers, data stewards, solution architects, and analytics translators. These AI teams collaborate to develop and implement AI solutions across different manufacturing functions.
Understanding the minimum level of governance and responsibility is key to implementing AI systems successfully. As a company transitions more processes to AI systems, companies need to define data ownership, security of the AI model, fairness and explain-ability of the results, and the ethics and regulation of the AI model. Identifying the owner of AI systems ensure that the results are implemented correctly and fairly.
Editor's Pick: The Journey of Additive Manufacturing and Artificial Intelligence
Lastly, as with many new systems, the culture can make or break AI adoption. Educating your workforce into trusting the data and AI algorithms will help create a higher level of acceptance. Business leaders also need to answer concerns of AI replacing workers, ease fears of risks, and spell out clearly AI’s limitations. Hopefully, if done correctly, creating a workforce properly educated about the power of AI may spark creativity and excitement.
Carlos M. González is special projects manager.
Adnan Masood, Ph.D. and chief architect of artificial intelligence and machine learning at UST, recently listed all the technologies within manufacturing that look to benefit from AI.
“Beyond hypes and fads, AI works because it amasses significant benefits for the manufacturing sector, such as enabling smart production, developing predictive and preventative maintenance, offering supply chain optimization, improved safety, product development, and optimization, facilitating AR/VR (Augmented and Virtual Reality), cost reduction, quality assurance and enabling green operations (energy management), to name a few,” he wrote for IntelligentCio.com.
With AI being able to impact so many different aspects, it isn’t easy to understand how it will transform manufacturing processes realistically.
Recommended for You: AI and Optimization: A Meeting of the Minds
PwC released “An Introduction to Implementing AI in Manufacturing” in 2020 to help lay out how AI can be deployed across different companies. According to PWC, there are four types of AI:
- Assisted Intelligence: AI systems that aid humans in decision-making situations. These systems are hardwired and do not learn from their interactions.
- Augmented Intelligence: AI systems that augment rather than aid human decision-making and evolve with every interaction with humans and the environment.
- Automation: Automating both manual and cognitive tasks that are routine or non-routine. The AI does not augment or evolve the task but only simply automates the process.
- Autonomous Intelligence: AI systems that can automate and adapt a task based on different scenarios without human assistance.
Implementing AI systems is a fundamental change to an organization’s manufacturing process. It is not as simple as buying a software package and installing it on your computer network. It requires investment and careful planning, from the operational tools to data acquisition and even the types of skilled workers a company hires. According to PWC, there are six building blocks for implementing AI successfully regardless of where you are in the AI process.
Business Applications
The main starting point for an AI strategy is to understand the near-, mid-, and long-term goals. Businesses should group their use cases across all operations into these categories based on function and priority. Identifying low-hanging fruit and obtaining early success will help determine the larger potential of AI and how to plan a road path toward broader implementation.
You May Also Like: 6 AI and Machine Learning Tools for Engineers
Data
The collection of data is key to driving AI systems. However, the key is that data be reliable and accurate, not just a mass collection of information, an aspect of data analysis that plagued early Industry 4.0 systems. The benefit of AI and machine learning is that when data is collected properly, the software can analyze and interpret the data for human workers. Advanced systems can even provide relevant input when making decisions. Large data-management systems require proper cataloging and lineage for tracking purposes and accessibility. Manufacturers need to understand the volume and velocity of data to map their manufacturing processes properly.
Technology Investment
PwC points out that the technology for AI systems is rapidly evolving and has yet to reach a mature state. This will cause companies to hesitate from investing in AI systems. However, PwC argues that investing in “functional reference architecture”—the very basics of AI systems—will help companies gain footing and allow them to evolve with AI systems. Some of these essential systems include data management software and cloud-computing services.
Talent and Skills
When Industry 4.0 processes started to make waves among manufacturing companies, the lack of data scientists to support advanced manufacturing systems impacted its growth. Since then, many organizations have hired data scientists to help make sense of the data collected, but AI systems will require an entirely new team of engineers. According to the PWC, data scientists need to be part of a team that includes data engineers, data stewards, solution architects, and analytics translators. These AI teams collaborate to develop and implement AI solutions across different manufacturing functions.
Processes
Understanding the minimum level of governance and responsibility is key to implementing AI systems successfully. As a company transitions more processes to AI systems, companies need to define data ownership, security of the AI model, fairness and explain-ability of the results, and the ethics and regulation of the AI model. Identifying the owner of AI systems ensure that the results are implemented correctly and fairly.
Editor's Pick: The Journey of Additive Manufacturing and Artificial Intelligence
Culture
Lastly, as with many new systems, the culture can make or break AI adoption. Educating your workforce into trusting the data and AI algorithms will help create a higher level of acceptance. Business leaders also need to answer concerns of AI replacing workers, ease fears of risks, and spell out clearly AI’s limitations. Hopefully, if done correctly, creating a workforce properly educated about the power of AI may spark creativity and excitement.
Carlos M. González is special projects manager.