Inspector Watson Does Quality Control
Inspector Watson Does Quality Control
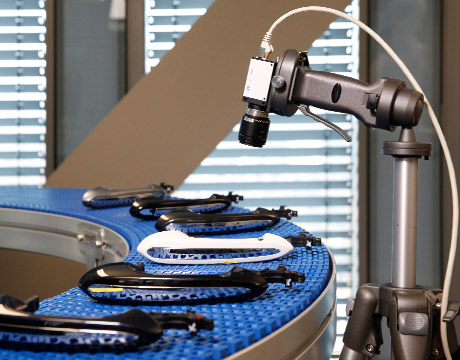
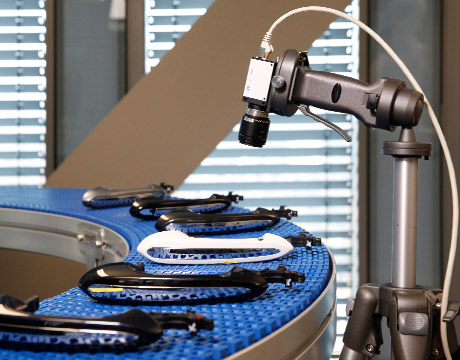
The new Cognitive Visual Inspection solution enables manufacturers to improve productivity of their manufacturing and assembly processes. Image: IBM
Artificial intelligence makes our phones more responsive, drives our cars, and humiliates us at our favorite games. And that’s just the beginning. The technology will soon worm its way into every aspect of life—including the world of manufacturing.
Thanks to the deep learning capabilities of IBM’s Watson-based Cognitive Visual Inspection, humans may no longer need to stand next to assembly lines, deciding which products are deemed acceptable and which are not.
Watson, IBM’s response to the Turing test, a method of probing a machine’s intelligence, answers questions in a Q & A format. In 2011, it appeared on “Jeopardy!”, trounced its human competitors, and earned $1 million dollars. Since then, it’s been put to use as the engine behind software that will help doctors diagnose, shoppers shop, and educators educate, among other things. It also powers IBM’s CVI on the assembly line. By analyzing images fed to it from ultra-high definition cameras, Watson is now helping manufacturers flag defective parts.
To identify a bad part, model managers and data scientists compile a library of images representing both ‘good’ and ‘not good’ parts. They feed those images to the Visual Inspection system to train Watson to recognize the ‘not good’ parts, says Jiani Zhang, program director for IBM Watson Internet of Things. Characteristics of ‘not good’ could include missing components on a circuit board, paint bubbles, surface scratches, corrosion, or mislabeled items.
But Watson doesn’t just refer to a static catalog of potential defects. As it analyzes products, Watson gets smarter, learning more and more about what’s good and what’s bad. To do so, it needs a pair of eyes of the blood and flesh kind.
“In order to create an efficient process, an inspection supervisor is required to set a predetermined threshold to determine which products need to be manually inspected,” says Zhang. “For example, they might require images captured by the factory floor camera that are only an 80 percent match with the corresponding image of a defect in the image library (to) be flagged for review. This metric allows inspectors to review items with human expertise to identify new types of defects and ensure efficiency on the manufacturing floor.”
In a typical situation, an inspector supervisor will only have to look at the pieces that Watson has flagged to classify them as defective or usable. The inspector supervisor will then make a classification determination and inform Watson. That data can be uploaded to the cloud and used in multiple locations.
Almost any manufactured part could benefit from Watson’s gaze.
“Any industry where manufacturing flaws can be detected visually are suitable for the system,” says Zhang. Products that are visually continuous, such as sheet metal, may still need the human eye.
The main challenges in making the Cognitive Visual Inspection inspector a reality had to do with the photographic end of the system, Zhang says.
“Camera-shooting environments have to be controlled so that images taken are consistent across the line for optimal accuracy and results,” she says. “We need to prevent issues such as glare and other lighting challenges to get an accurate and clear picture.” IBM is currently working with camera and robotics makers to create the most consistent, high fidelity images possible for Watson’s interpretation.
With them, guesswork and human error can be further removed from the conveyor belt.
Any industry where manufacturing flaws can be detected visually is suitable for the systemJiani Zhang, Program Director, IBM Watson Internet of Things