Building Dexterity into Robotic Hands
Building Dexterity into Robotic Hands
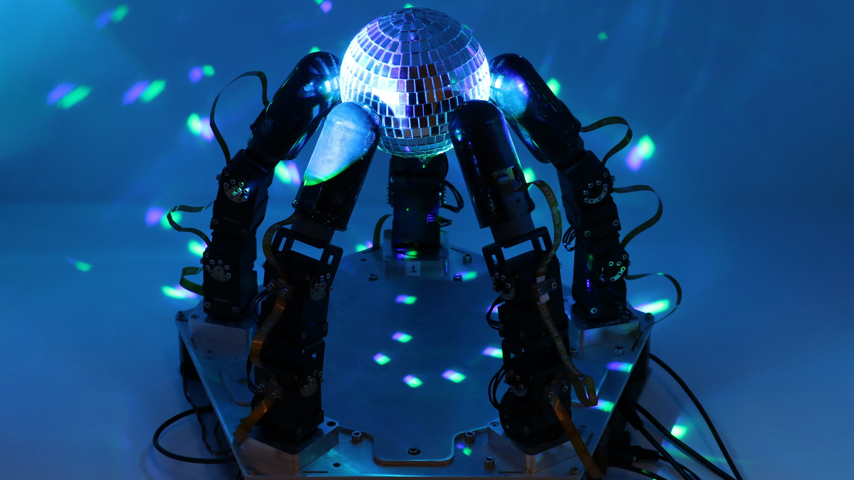
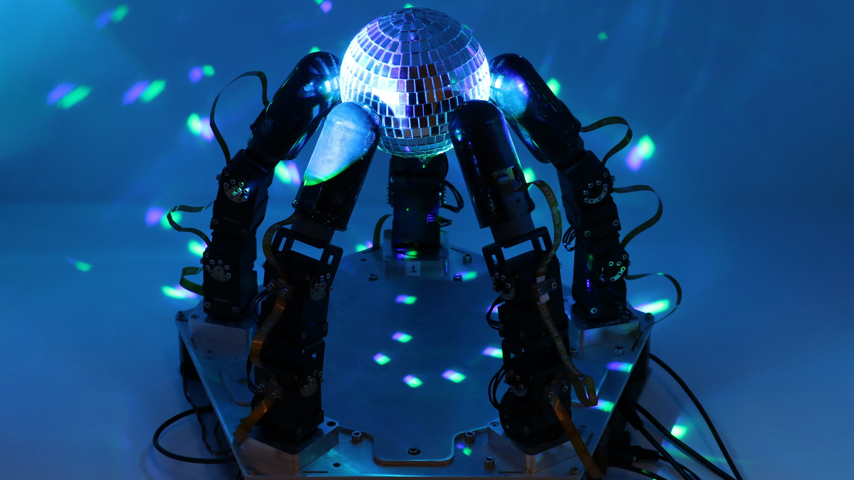
Columbia researchers used new methods for motor learning to build fingers that can grasp and rotate an object.
What looks a bit like a thick-tentacled sea anemone waves its appendages to and fro, but the digits aren’t blindly trying to ensnare prey. Instead, they’re working in concert to grasp an oddly shaped block with numerous surfaces and rotate it, like a human hand manipulating one of those silvery exercise balls. Like those of a human, the fingers of this robotic hand work on touch alone, without cameras. Even in darkness, the digits easily feel their way around an object, squeezing just tightly enough on one surface and loosening its clutch on another so that it can twirl the block clockwise and counterclockwise, or rotate it top to bottom.
This so-called “highly dexterous robot hand” is among the latest inventions out of Columbia University’s Robotic Manipulation and Mobility Lab. The multifaceted team responsible for creating the hand is led by Matei Ciocarlie, an associate professor of mechanical engineering. He’s been working to create these dexterous devices since 2015 with the end goal of putting them onto robots for assisting in the home, or for manufacturing, logistics, and materials handling.
Dexterity has been a longstanding goal of robotics developers, but with only limited success. Grippers and suction devices are most commonly used to pick up and put down items, but robotic assembly has been slower to develop. More advanced sensors and machine learning algorithms are helping the work to advance.
Those fingers, which for now are mounted on a flat platform rather than human palm-like base, have come a long way in eight years, when the team initially used piezoelectric sensors for touch. In the new iteration, each of the fingers has a flexible reflective membrane beneath its 7-mm to 8-mm skin. Beneath that is an array of LED lights and phototdiodes. Each of the lights is cycled on and off for less than a millisecond, and the photodiodes record the reflection of the light off the inner membrane.
“When a finger touches anything, it bends the ‘skin’ and changes how the light bounces around,” which then tells the digit how tightly it’s gripping the object, and exactly what part of the finger’s surface is touching the object, said Ciocarlie. It’s a system that behaves much like our own nerves and skin, providing a very localized sense of touch. It is sensitive to under 1 mm accuracy all over, even on the backs of the joints, something that eluded robotics until now, he said.
Become a Member: How to Join ASME
Each clear finger is usually encased in a black cover, but when it’s not, “We call it the disco finger,” he joked. “If robotics doesn’t work out, it can be used for decoration.”
The device is about four times as large as an average human hand, said Gagan Khandate, a Ph.D. student in the computer science school who led this work. Its current size makes it almost immediately applicable in packaging and assembly, he said. Making it smaller, closer to human-sized, could be difficult, but a miniaturized hand might more easily be able to handle challenging, slender shapes like pencils. Once the hands are mounted on robotic limbs, "There’s a lot of things we can achieve with just an arm, maybe two arms, without being attached to a moving platform,” he said.
The physical attributes of the fingers and hand look impressive, but Ciocarlie and Khandate said the real challenge was training the devices to become dexterous. “Robotics is a system science,” Ciocarlie explained. “Especially when combining hardware and software, it’s tricky,” particularly when teaching the device to learn the hard motor skill of simultaneously grasping and rotating an object.
The team used a machine learning technique called reinforcement learning, in which a robot learns from trial and error, rather than pulls answers from a database of information. “It’s not shown the task; it tries to do it, and gets feedback,” said Ciocarlie. It then saves that knowledge for next time."
Take Our Quiz: Robots Inspired by Nature
Reinforcement learning works for very complex tasks, but this often takes a very long time. To speed up the process, the robotic components were trained in simulators — thousands can be trained in parallel — which allowed them to gain a year’s worth of experience in just an hour and a half.
The hand had to learn proper finger gaiting, how to reposition each finger as together they hold and rotate the object. To this end, the team used sampling based motion planning, a method to compute a sequence of moves.
Collecting all this data was one of the greatest challenges on this project, said Khandate. But the evolution of machine learning, “especially in robotics, is quite impactful. It allows us to achieve complex skills we hadn’t been able to do previously,” he said.
In July, Ciocarlie will present the team’s work at the Robotics: Science and Systems conference in Korea: “What I’m going to highlight is first, from an applications perspective, these dexterous tasks: finger gaiting with a secure grasp. Then I’ll highlight learning and sampling based motion planning. It’s the combination of these three that makes it tick,” he said.
Eydie Cubarrubia is an independent editor and writer in New York City.