Avoiding Existential Crises on the Virtual Battlefield
Avoiding Existential Crises on the Virtual Battlefield
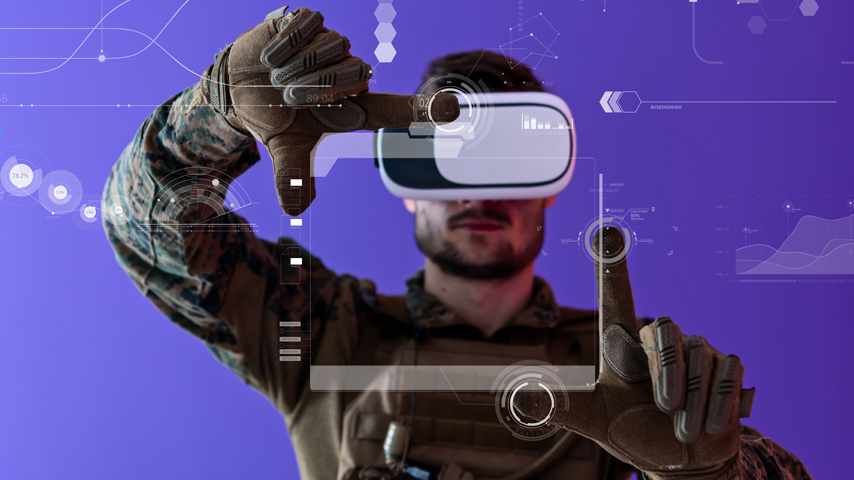
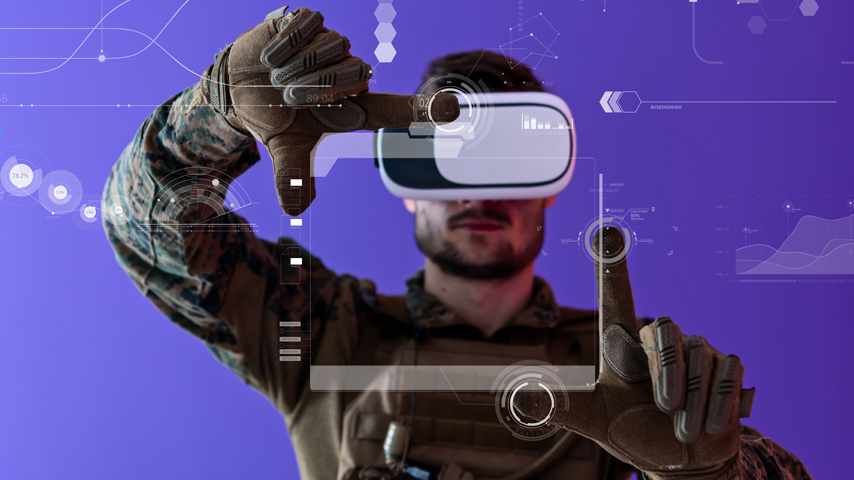
A new algorithm lets anyone immersed in a 3D model know the value of any piece of data.
In any military operation, it’s as important to know the terrain as it is to know the enemy. Soldiers who have had a chance to run through an exercise before the real thing are going to have an edge on the battlefield. But the battlefields of an active or potential conflict, be they desert, field, or city, are not the best places for pounding out practice, so the military has been relying more and more on virtual simulations.
But the data used to build those simulated battlegrounds can have inaccuracies. A pole or a hill might not have the dimensions that appear in the model, and soldiers who assume that what they see in a simulation will be exactly what they find in the real world are putting themselves at risk. Now researchers at The Ohio State University’s College of Engineering, with funding from the Office of Naval Research, have found a solution: Tell the user in the simulation just how likely it is for an object that appears there to be the same size on the actual battlefield.
Become a Member: How to Join ASME
In the most obvious example, a combatant might use some edifice to hide behind, confident that he’s not visible from the other side. “The 3D data accuracy is not something that you are aware of. It could be, plus-minus one meter or it could be plus-minus half a meter,” said Rongjun Qin, a professor of geodetic engineering at the university and principal investigator on the project. “So what we’re going to do here is say, ‘Hey, I know this area photograph has some limitations, but we want to let you know which part of the points are more accurate than other ones.’”
The images used to build a 3D scenario come from planes, drones, and other sources. Sometimes those images are just too low-resolution to create an accurate 3D construct. Sometimes smooth surfaces and glass create noise that limits accuracy. Qin was able to gauge that accuracy by comparing pixels, which represent a certain area on the ground, to scale data as determined by the camera focal length and the flight height. “You can use that information to get the ‘ground sampling distance,’ which would serve as a basic unit to propagate all the metrics,” he explained.
More for You: Improved GPS Will Put Us in Our Place
With Qin’s code in place, a soldier running through the virtual streets, say, of a war zone will see numbers in a rectangle on every object, indicating its likely maximum and minimum size. That same code will show which areas of a virtual battlefield are most likely to be inaccurate so that a drone, or some other image-collecting device, could be used to subsequently fill in the details.
But the battlefield is not the only place where knowing how much a bit of trusted 3D data would be useful. For example, logging companies use 3D data to estimate the yield of a forest, hydrologists examine such data to make flood predictions, and anyone building a digital twin for a city needs this kind of information. Similarly, the accuracy of the data is also important for autonomous driving, especially in places with heavy traffic and narrow streets. And, of course, there are many other situations where knowing the accuracy of any image or piece of data would greatly refine the model and its usefulness.
“In most cases, when people are using 3D data, they just trust it,” said Qin. “But there’s a lot of uncertainty when the numbers of a simulation don’t really match the real world. If you have that as part of the data standard, you can actually plus-minus that error bar for people to make better decisions.”
Michael Abrams is a technology writer in Westfield, N.J.
But the data used to build those simulated battlegrounds can have inaccuracies. A pole or a hill might not have the dimensions that appear in the model, and soldiers who assume that what they see in a simulation will be exactly what they find in the real world are putting themselves at risk. Now researchers at The Ohio State University’s College of Engineering, with funding from the Office of Naval Research, have found a solution: Tell the user in the simulation just how likely it is for an object that appears there to be the same size on the actual battlefield.
Become a Member: How to Join ASME
In the most obvious example, a combatant might use some edifice to hide behind, confident that he’s not visible from the other side. “The 3D data accuracy is not something that you are aware of. It could be, plus-minus one meter or it could be plus-minus half a meter,” said Rongjun Qin, a professor of geodetic engineering at the university and principal investigator on the project. “So what we’re going to do here is say, ‘Hey, I know this area photograph has some limitations, but we want to let you know which part of the points are more accurate than other ones.’”
The images used to build a 3D scenario come from planes, drones, and other sources. Sometimes those images are just too low-resolution to create an accurate 3D construct. Sometimes smooth surfaces and glass create noise that limits accuracy. Qin was able to gauge that accuracy by comparing pixels, which represent a certain area on the ground, to scale data as determined by the camera focal length and the flight height. “You can use that information to get the ‘ground sampling distance,’ which would serve as a basic unit to propagate all the metrics,” he explained.
More for You: Improved GPS Will Put Us in Our Place
With Qin’s code in place, a soldier running through the virtual streets, say, of a war zone will see numbers in a rectangle on every object, indicating its likely maximum and minimum size. That same code will show which areas of a virtual battlefield are most likely to be inaccurate so that a drone, or some other image-collecting device, could be used to subsequently fill in the details.
But the battlefield is not the only place where knowing how much a bit of trusted 3D data would be useful. For example, logging companies use 3D data to estimate the yield of a forest, hydrologists examine such data to make flood predictions, and anyone building a digital twin for a city needs this kind of information. Similarly, the accuracy of the data is also important for autonomous driving, especially in places with heavy traffic and narrow streets. And, of course, there are many other situations where knowing the accuracy of any image or piece of data would greatly refine the model and its usefulness.
“In most cases, when people are using 3D data, they just trust it,” said Qin. “But there’s a lot of uncertainty when the numbers of a simulation don’t really match the real world. If you have that as part of the data standard, you can actually plus-minus that error bar for people to make better decisions.”
Michael Abrams is a technology writer in Westfield, N.J.