The Secret Life of the Spine
The Secret Life of the Spine
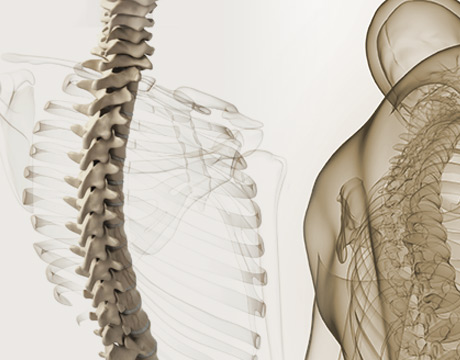
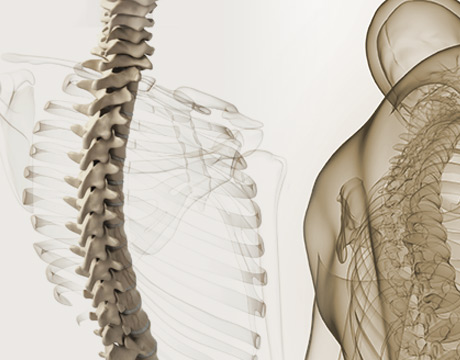
Lower back pain is the second most common reason people visit their physicians and the world’s leading cause of disability. In the United States alone, back pain accounts for more than 100 million annual lost work days and $90 billion in treatment costs. That is as much as we spend fighting cancer.
The culprit is usually the spine.
And despite all the problems spines cause, we know shockingly little about them. Physicians fail to provide a precise diagnosis for 80 to 90 percent of lower back pain cases. MRI images and computed tomography scans provide useful information a paltry 10 to 15 percent of the time. Diagnoses are mostly subjective, treatments usually trial and error. Surgery fails more than half the time, and follow-up surgeries have an even lower rate of success.
For the past three decades, the Spine Research Institute at Ohio State University has sought to unlock the secrets of the spine. We did this by measuring spines under different loads and building models accurate enough to simulate their behavior. We use these models to discover more about the causes of back and neck pain.
Our biomechanical models are not mere academic exercises. We have used them to redesign tasks to reduce the risk of spinal injuries on assembly lines and in such professions as nursing. Our most recent work seeks to provide physicians and surgeons with more precise ways to diagnose and treat back pain.
To get there, however, our biomechanical models had to come a long, long way.
Muscles and Loads
The first attempts to model the spine yielded generic models based on moments, or force times distance. They treated the spine as a fulcrum, the center of our biological seesaw. One side of the seesaw carries the weight of our body and anything we lift or move. On the other side, muscles anchored to our spine counterbalance the load.
To create these early models, engineers used inverse dynamics. Working backwards, they noted the static force on one side of the seesaw and calculated the counterbalancing torque applied by the spine.
As an athlete who played basketball in college, I knew this approach had to be wrong. Totally wrong. This is not how muscles respond to force.
Instead of a single counterbalancing torque, the muscles in our back, torso, arms, and legs engage in a constant tug of war with one another. They have to, because the loads on our spines are rarely static. We constantly bend, twist, lift, run, jump, and move.
Moreover, every spine is as unique as our teeth or face. For example, while the dictionary definition of the lumbar vertebrae is the five vertebrae between the pelvis and rib cage, our own imaging studies showed that a surprisingly large number of people have six rather than five lumbar vertebrae. (This has led to failed operations because surgeons failed to verify the location of a vertebra by counting from both the top and bottom of the spine.)
Even when spines have the same number of vertebrae, they behave differently. Their vertebrae come in all sizes, densities, and shapes. Each one handles loads differently. The same is true of muscles. Not only does strength vary from person to person, but each of us uses our muscles differently.
LeBron James, for example, recruits his muscles a lot differently than I do.
A simple mechanical model cannot possibly predict spinal behavior. That is why, more than 25 years ago, we made a strategic decision to develop biologically assisted models. To do this, we needed to understand the construction of the spine (fairly straightforward), and also how muscles—down to individual vectors of force within a muscle—worked with one another to generate force.
That meant finding ways to tease out how muscles generate force.
One way was electromyography (EMG), which reads the body’s electrical signals to tell us which muscles act together and how strongly they contract. We took EMG readings while subjects did specific tasks, then calibrated that data with the force exerted by those muscles.
;custompagebreak;We spent years doing this experimentally. Equally important, we wanted to capture those forces dynamically, rather than settle for the static measurements used by previous models.
We developed a 10-foot-tall dynamometer that pivoted to match the twisting, turning, and bending of the lower back so we could measure torque. We asked people to push, pull, lift, move, and stand on force plates to measure force. We outfit our subjects with cables to goniometers that measured the angles of their spines as they moved in space.
This let us triangulate where the spine was relative to the force plate. We also noted the moments of force. This let us work backward from the force on the force plates, and let us make predictions about the moments on the spine.
It was slow work, and the first models were not very good. We rarely achieved more than 60 percent agreement of predicted and measured forces. Rapidly advancing technology helped us raise our accuracy to greater than 90 percent.
One important advance was the introduction of graphics-ready Windows computers. Before them, we would make measurements, then spend the next few days crunching long columns of numbers and generating graphs.
Windows let us plot and compare our predictions with experimental measurements. If the predicted moments deviated from the observed data, we could reexamine the behavior of the muscles and change the model on the spot. Then we could run the experiment again and see if the model did better. Before Windows, that process would take days or even weeks.
We also learned to use magnetic resonance imaging and computerized tomography scans to study muscle size, location, and lines of action. We could focus down to individual vectors of force within a muscle, and use what we learned to predict how they would generate lines of action within groups of muscles.
Imaging let us see how close muscles were to the center of the spine, which determined their mechanical advantage and ability to load the spine. This proved part of the secret sauce that let us leap from EMG data to an accurate picture of the forces placed on the spine.
Into the workplace
By the late 1990s, we were ready to take our models out into the world.
Early on, we teamed up with the Ohio Bureau of Workers Compensation. It was struggling with claims from factory workers who injured their backs lifting. While the National Institute of Safety and Health had developed safe lifting guidelines, factory owners and managers had problems working the formula.
There were also issues with the formula. Many early spine models were based on quasi-static data. This involves taking measurements of moments and forces at various stages of a task, and assuming they are equivalent to the dynamic forces generated during the same task.
We knew that wasn’t the case. To model the dynamic loads, we built a mockup assembly line and measured the forces involved in each task. Then we entered them into our model to see how those actions loaded the spine.
The first thing we found was that the dynamic forces on the spine were higher—sometimes as much as 70 or 80 percent higher—than quasi-static models predicted.
We also found that body motion often mattered as much as weight and sometimes even more. For example, the difference between lifting 25 pounds or 50 pounds from the same position was miniscule when compared to the difference between lifting a load from different positions (such as floor versus waist level).
Using this information, we based our safety guidelines on the height of the load, how far from the body a worker held it, and how much he or she had to twist while holding it. The Ohio Bureau of Workers Compensation used our data to create a web app that yields safety limits with only a few inputs. The app still draws about 2,000 queries each month.
We began teaching short courses in quantitative ergonomics to share what we had learned about back safety. A Honda manager who took one of the courses invited us to meet the company’s leadership.
In the early 2000s, Honda was experiencing lots of musculoskeletal problems on a door assembly line. We looked at injury records, identified the top 10 riskiest jobs, and recreated the work conditions in our lab so we could learn what made those jobs so risky. We wired up workers and monitored their lifts, holds, and twists. Once again, we used that data to drive our models and see how those forces acted on the spine.
;custompagebreak;We were looking for risky conditions and we found them. In some cases, our recommendations were obvious, like rearranging the workplace so that workers never had to lift anything from the floor. We also proposed relocating racks to reduce twisting, and redesigning carts to minimize pushing forces.
Then—nothing. Honda did not get back to us. We assumed they were unhappy with our work. One year later, they returned. Some managers had initially resisted changing their manufacturing process, but were swayed by our hard data on risks and spinal loading.
Honda spent $89,000 to implement five of our 10 suggestions. The reduced injury costs and increased work efficiency saved the company $360,000 the first year at five workstations. Over the next five years, Honda would save $1.8 million by rolling out a single workstation solution in other plants.
Honda wanted to go further. It became a founding member of Ohio State’s Center for Occupational Health in Automotive Manufacturing, or COHAM.
COHAM’s 8,000-square-foot space looks like a manufacturing facility. It includes ceiling-mounted cranes and a rotating auto conveyor. The lab has the ability to raise, lower, and rotate cars, as well as provide workers with different types of assistance. It enables us to quantify things that have never been measured before.
COHAM also benefits from new technology, such as motion-capture technologies that use optical, inertial, and infrared sensors instead of cables. By applying the lab’s findings, Honda reduced musculoskeletal injury rates by 70 percent throughout its North American factories.
The same approach has also begun to make a difference in the occupation most prone to back injuries—nursing. Nurses are constantly lifting and manipulating patients. They move them in or out of beds, roll them over to empty bed pans, give them showers, take them to commodes, or work with them in physical therapy. As a consequence, nurses suffer an epidemic of back problems. By the mid-1990s, the State of Washington was debating whether to require that patients could be lifted only by groups of two or more nurses.
On the face of it, this made sense. Our research showed that the issue was not so clear-cut.
Individuals could generate dangerous compressive loads on the spine when lifting patients alone. Yet when two nurses worked together, they typically stood too far away from the patient to get their legs under the load, so their spine was bent when they began to lift. This put enormous shear loads on the spine. In fact, lifting together was often just as bad as working alone.
This small study made a big impact. Washington enacted a zero-lift policy, and required nurses to use patient-handling devices. While some nurses initially resisted these recommendations, the hard data behind our recommendations helped mobilize many more to demand assistive devices.
Many hospitals complied. Based on our studies, the Veterans Administration invested $280 million at its national network of care centers. A Stanford University study estimates that it is saving many times that amount by reducing disability costs and improving medical care.
How Bad Does It Hurt?
Going forward, our models could help doctors diagnose and treat back pain. Until now, there has been no objective way to quantify the extent of an ailment or how well a treatment is working. Instead doctors typically ask patients about activities of daily living, such as whether they have trouble getting out of bed or dressing.
They may also ask patients to rate their pain on a scale of one to 10 as they bend, twist, and stretch. Experienced physicians will quickly pick out small hiccups in those motions, unexpected variations in movement that look unnatural.
Yet while humans are very good at assessing distances, they are terrible at estimating changes in velocity and acceleration. Yet these small variations in body motion provide powerful clues to the nature of back problems. A combination of movement sensors and models might help doctors capture this information—and how it changes over time—and use it for more accurate diagnoses.
We also believe spine models will become important tools for surgeons. For the past 30 years, the Spine Research Institute has sought to discover the causal pathways of back pain. We knew our answer started with a force, and we turned to biomechanics to understand how that force creates muscular or structural problems in the spine.
While we can solve muscular problems with physical therapy, structural problems usually require surgery. Yet spinal surgeries are successful less than half the time because it is so difficult to quantify what is wrong with the spine.
Spinal models can provide that type of information. We can individualize them by modifying our general models with information about individuals captured by video analysis and gathered from MRI and CT scans.
These models generate animations that show how the spine reacts to force as it moves in space. They highlight where—and why—discs bulge and shifting sections of the vertebrae impinge on nerves. They automatically pinpoint structural overloads.
This is information surgeons have never had before. They will be able to see the source of the problems previously hidden from them. Equally important, they can test surgical strategies virtually to see which ones provide immediate relief without long-term complications.
It would, quite simply, revolutionize spinal surgery.
This is because spines are like teeth. We all have teeth, but some are large, small, straight or crooked. The spine is the same way. We all have one, but it is unique. The devil is always in the details. Dentists navigate those details by taking an X-ray, checking our bite, and poking and prodding our teeth and gums.
Spinal surgeons cannot do anything like that, at least not physically. Solid models give them a way to poke and prod our spines virtually. They can add a screw here or a titanium rod there. They can change their size, shape, and material properties, or perhaps add a disc or two.
The hardware is the easy part. Knowing where to put those parts is difficult. This is why we are working with Ohio State Medical Center’s spine surgeons to apply our models to surgical decision-making and planning.
It has taken more than 30 years of research, but we finally have tools that give the ability to see back problems more clearly. ME
William S. Marras is executive director of the Spine Research Institute and the Honda Chair professor at Ohio State University in Columbus. He is also president-elect of the Human Factors and Ergonomics Society.
Little is understood about a critical body structure; engineers are changing that.