Nano Recall
Nano Recall
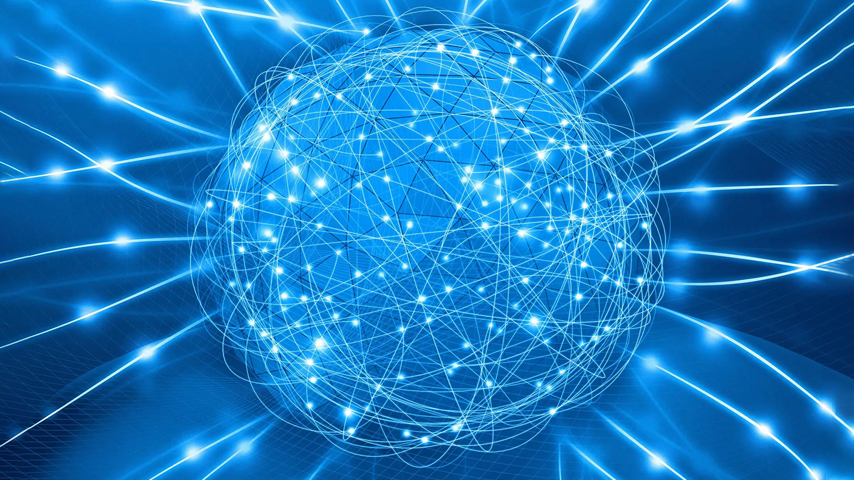
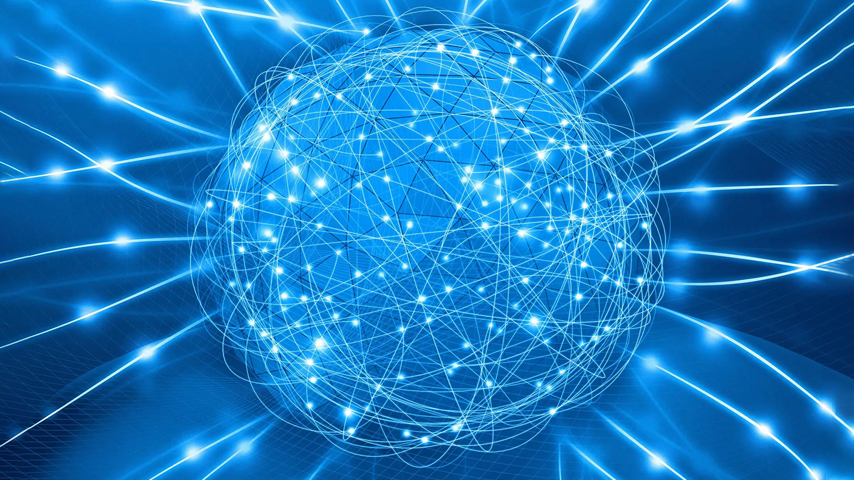
A network of tiny wires forms memories that, like our own, stick with repetition.
Take a handful of nanowires, drop them on a surface like so many pick-up sticks, and they’ll self-assemble into something that looks remarkably like a network of neurons. Why? No one knows.
“You know, weird stuff happens at the nanoscale that we don’t fully understand,” said Alon Loeffler, recently a doctoral student in the physics department of the University of Sydney, and now a synthetic biological intelligence post-doctoral scientist at Cortical Labs in Melbourne, Australia, by way of explanation. What’s weirder is that Loeffler’s randomly tossed nanowires are capable of remembering things.
This nano memory is not just the permanent kind, like a file on a hard drive, but the kind that decays over time and gets reinforced with learning. In other words, a network of nanowires is strangely brain-like. By training and studying such networks, Loeffler is providing a new understanding of what might be going on in the flesh and blood networks of our brains, while simultaneously paving the way for faster and more efficient AI systems.
The nanowires Loeffler tossed together are made of highly conductive silver, coated in an insulating plastic polymer. Where those wires cross, the insulating layers create a gap—not unlike a synapse. When an electrical pulse is sent through the wires, it sometimes makes the jump from one wire to another at an intersection and sometimes it doesn’t.
Similar Reading: 5 Ways Nanotech Affects Everyday Life
“We get this weird kind of bridge formation or ion migration, piercing through that insulating layer,” said Loeffler. “All these unpredictable, nonlinear, cool kind of behaviors occurring at the nanoscale give rise to these activation functions at each crossover.”
Loeffler and his colleagues, Zdenka Kuncic, a professor of physics at the University of Sydney, and Adrian Diaz-Alvarez, a researcher at Japan’s National Institute for Materials Science, hooked up the network to source electrodes on one side and drain electrodes on the other. When he turned on the juice, it took a seemingly random walk from input to output—the path was of its own choosing, not dictated by the researcher. But once that first path was formed, the conductance along it increased and the current flowed much more easily to the same places with subsequent pulses.
Loeffler wanted to see if he could tune the network so that a current introduced to one input would end up at an output of his choosing. The pathway didn’t matter. “We can’t physically go in and control any of the junctions or the wires without breaking them,” said Loeffler. “We don’t even know where those junctions are in the system most of the time because it’s so hard to see.”
To train the pathway to go to one output they simply closed off the other options, forcing the current to flow from point A to point B. Then they opened up all the outputs to see if the network retained those pathways. If it did, they considered the memory made, and if it didn’t, they would increase the voltage slightly on the non-target drains to create a path of least resistance to the target. (A voltage that's too high can burn the wires.) With multiple iterations, they eventually created lasting memories.
But, like most short-term memories, they don’t last forever, and are dependent on reinforcement.
Become A Member: How to Join ASME
“When the bridges are formed at the junction level, they’re formed, and then, when you stop sending electrical signals through it, they’re still formed,” said Loeffler. “What you get is a slow decay of that bridge, of those ions moving back into the original wire.” As long as those ions haven’t finished their retreat, electricity sent through the system again will cross those junctions much more quickly.
Memories in biological beings tend to stick around longer with repetition, as any musician, athlete, or cramming college student knows. To find out if the nano network retained its pathways when similarly reinforced, Loeffler and his colleagues tested it against psychology’s N-Back task. In that experiment, previously done with humans, subjects are given a pattern to remember followed by a number of interference patterns. Then they’re tested to see if they can remember the original pattern. The idea is to see how well a memory holds up to repeated interference.
Similarly, Loeffler had a target pattern that he sent to a specific output. Then he randomly sent different patterns, or pathways, to different outputs (seven in total, as there were seven drain electrodes) as interference patterns, before testing the first pathway.
“What we showed is that, without the reinforcement, it would dramatically drop off very, very quickly. It could only really remember two or three steps backward,” he said. “But when reinforcement was introduced, it was almost 100 percent. Every time. It didn’t matter how many different patterns interfered between.”
In the animal brain, there is a constant firing of neurons—even during deep sleep and under anesthesia. Recent research indicates that this continuous activity may be what maintains memory.
You Might Also Enjoy: Getting Closer to the Ability to 3D Print Tiny Structures
“We actually see this in our system,” said Loeffler. “If we feed in a very, very low voltage, the junctions will never decay. That might be analogous to this kind of resting-state firing in the brain.”
Put a network like that on a chip and it’s possible to reduce the power needs of AI and processing in general. The Von Neumann architecture that all computers work on continuously buses memory back and forth between the program and stored memory.
“It’s a huge bottleneck for traditional computing,” said Loeffler. “Neuromorphic systems and brain-inspired systems—and brains and biological systems—don't have that problem. We have this processing and memory occurring in the same place simultaneously. So, you don’t have latency, you don’t have that bottleneck.”
Though the research might someday lead to a superior neuromorphic chip—as opposed to the highly dedicated ones being produced by Intel, IBM, TrueNorth, and others—Loeffler was personally driven to understand the nature of thoughts, memories, and consciousness.
“If we can replicate these behaviors in a non-invasive way—you know, without having to cut into someone’s head and poke around—we may better understand the human brain,” he said
Michael Abrams is a technology writer in Westfield, N.J.
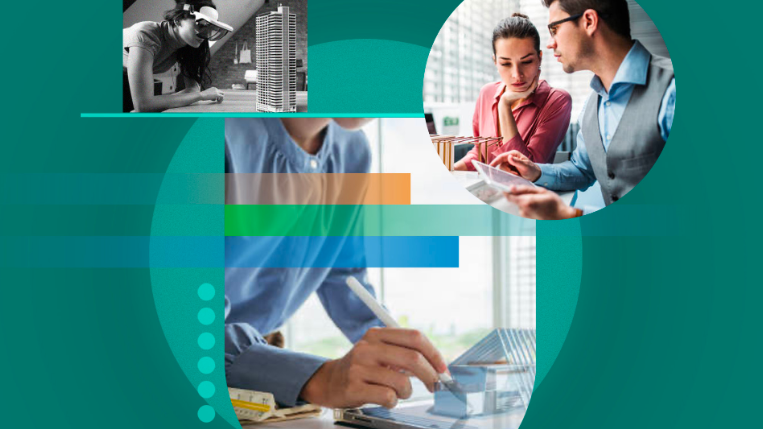